Image classification method based on CNN fragmentation multi-scale feature fusion
A technology of multi-scale features and classification methods, applied in the field of computer vision, to achieve the effect of reducing the amount of parameters
- Summary
- Abstract
- Description
- Claims
- Application Information
AI Technical Summary
Problems solved by technology
Method used
Image
Examples
Embodiment Construction
[0071] The present invention will be described in detail below in conjunction with the accompanying drawings and specific embodiments.
[0072] The image classification method based on CNN-based multi-scale feature fusion of slices of the present invention, the specific process is as follows figure 1 Shown: the method of the present invention is described below with the cifar-10 and cifar-100 public image data sets as an example.
[0073] Step 1: Preparation of image training set
[0074] Download the cifar-10 and cifar-100 image datasets online. The cifar-10 dataset consists of 60,000 32x32 color images of 10 classes, Figure 6 is the specific content of its 10 categories; each category contains 5000 training images and 1000 test images. The cifar-100 data set also contains a total of 60,000 32x32 color images. The difference is that the cifar-100 data set contains 100 categories, each of which has 500 training pictures and 100 test pictures. Table 3 shows that its categor...
PUM
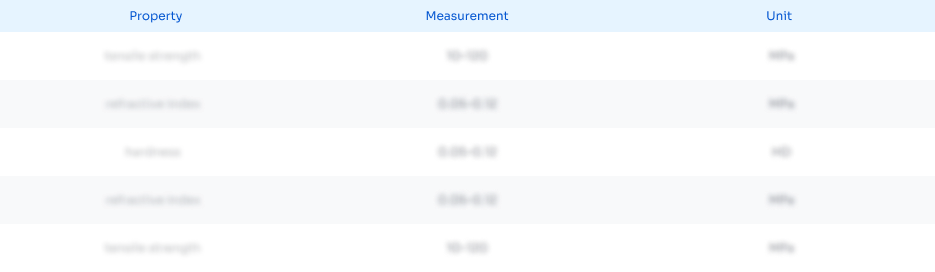
Abstract
Description
Claims
Application Information
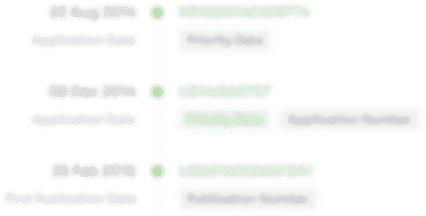
- R&D Engineer
- R&D Manager
- IP Professional
- Industry Leading Data Capabilities
- Powerful AI technology
- Patent DNA Extraction
Browse by: Latest US Patents, China's latest patents, Technical Efficacy Thesaurus, Application Domain, Technology Topic, Popular Technical Reports.
© 2024 PatSnap. All rights reserved.Legal|Privacy policy|Modern Slavery Act Transparency Statement|Sitemap|About US| Contact US: help@patsnap.com