Phase-dependent shared deep convolutional neural network speech enhancement method
A technology of deep convolution and neural network, applied in voice analysis, instruments, etc., can solve the problems of ignoring the verification of noise reduction results and the influence of voice phase information and phase information noise reduction effect, so as to expand the training data set and ensure the quality of voice quality effect
- Summary
- Abstract
- Description
- Claims
- Application Information
AI Technical Summary
Problems solved by technology
Method used
Image
Examples
Embodiment Construction
[0058] In order to express the purpose, technical solution and advantages of the invention more clearly, the present invention will be further described in detail below in conjunction with the accompanying drawings and specific implementation examples.
[0059] Such as figure 1 As shown, a phase-correlated shared deep convolutional neural network speech enhancement method, including:
[0060] Step 1, use the short-time Fourier transform to analyze the noisy speech data and the clean speech data in the time-frequency domain, and obtain the dual-channel time-spectrum features of the noisy speech data and the clean speech data, including the real part spectrum and the imaginary part spectrum , taking the dual-channel time-spectral features of noisy speech data as input and the dual-channel time-spectral features of clean speech data as supervised labels to construct training samples.
[0061] Select 500 voices of clean speakers, each voice duration is between 3 and 10s, select 3...
PUM
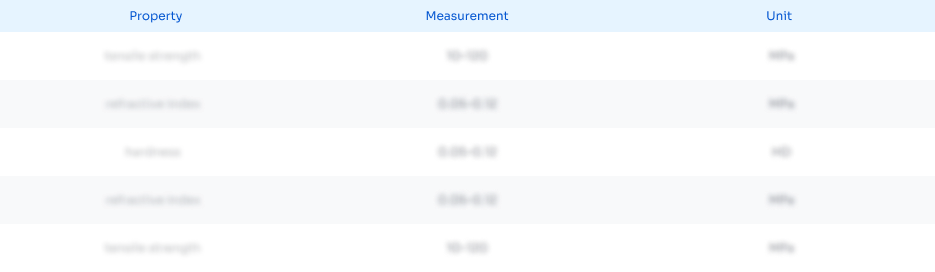
Abstract
Description
Claims
Application Information
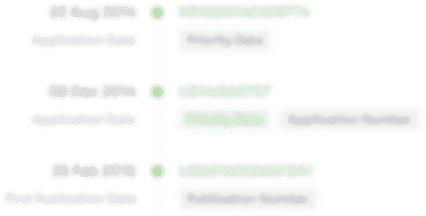
- R&D Engineer
- R&D Manager
- IP Professional
- Industry Leading Data Capabilities
- Powerful AI technology
- Patent DNA Extraction
Browse by: Latest US Patents, China's latest patents, Technical Efficacy Thesaurus, Application Domain, Technology Topic, Popular Technical Reports.
© 2024 PatSnap. All rights reserved.Legal|Privacy policy|Modern Slavery Act Transparency Statement|Sitemap|About US| Contact US: help@patsnap.com