Facial expression synthesis method based on generative adversarial network
A facial expression and generative technology, applied in the field of deep learning and image processing, can solve the problems of unnatural, unrealistic, low-resolution expressions, etc., and achieve the effect of convenient and intuitive method, vivid and real expression strength
- Summary
- Abstract
- Description
- Claims
- Application Information
AI Technical Summary
Problems solved by technology
Method used
Image
Examples
preparation example Construction
[0028] The present invention is based on the method for synthesizing facial expression images of a generative confrontation network, comprising the following steps:
[0029] Step 1, obtain the data of facial expressions;
[0030] Step 2, preprocessing the expression data set, first obtain the key point information of the face image, then cut the image into a uniform size according to the key point information, and divide it into training data and test data, and divide the training data according to different expression strengths Manually divided into different categories;
[0031] Step 3, constructing a generative confrontation network, and adding the discrimination information of expression strength and the sequence and correlation information between different strengths to the generative confrontation network;
[0032] Step 4, use the preprocessed expression data to train and test the generative confrontation network, adjust the parameters of the generative confrontation ne...
Embodiment 1
[0056] This embodiment takes CK+ (http: / / www.consortium.ri.cmu.edu / ckagree / ) and MUG (https: / / mug.ee.auth.gr / fed / ) data sets as examples for the present invention based on The face expression image synthesis method of generative confrontation network is researched, and the specific implementation steps are as follows:
[0057] Step 1. Download the facial expression sequence dataset from CK+ (http: / / www.consortium.ri.cmu.edu / ckagree / ) and MUG (https: / / mug.ee.auth.gr / fed / ) as Experimental data.
[0058] Step 2. After the data is acquired, it is preprocessed. In this embodiment, two expressions of happiness and surprise are taken as examples to study the proposed algorithm. In the CK+ data set, there are very few expression data, and there are only some expression labels. In order to make full use of the data, it is necessary to additionally classify the unclassified happy and surprised expressions. In the MUG data set, each subject's single expression contains some repeated se...
PUM
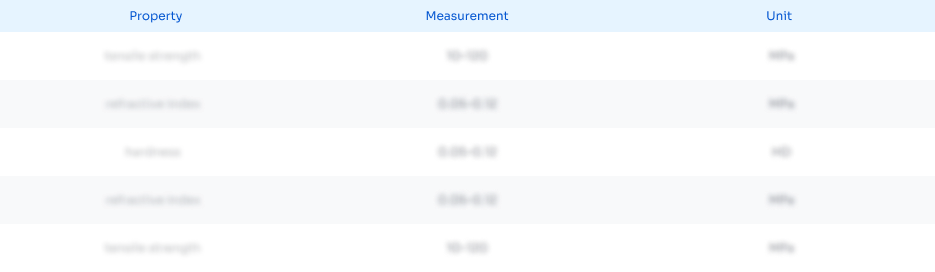
Abstract
Description
Claims
Application Information
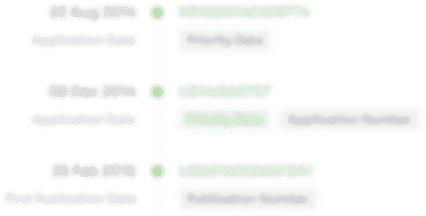
- Generate Ideas
- Intellectual Property
- Life Sciences
- Materials
- Tech Scout
- Unparalleled Data Quality
- Higher Quality Content
- 60% Fewer Hallucinations
Browse by: Latest US Patents, China's latest patents, Technical Efficacy Thesaurus, Application Domain, Technology Topic, Popular Technical Reports.
© 2025 PatSnap. All rights reserved.Legal|Privacy policy|Modern Slavery Act Transparency Statement|Sitemap|About US| Contact US: help@patsnap.com