Building short-term load prediction method based on ARIMA-LSTM combination model
A technology of short-term load forecasting and combined models, which is applied in forecasting, neural learning methods, biological neural network models, etc., can solve the problems of inaccuracy and single precision of building short-term load forecasting, and achieve the effect of improving forecasting accuracy and convergence speed
- Summary
- Abstract
- Description
- Claims
- Application Information
AI Technical Summary
Problems solved by technology
Method used
Image
Examples
Embodiment 1
[0065] Reference figure 1 with figure 2 , Provides a schematic diagram of the overall structure of a short-term building load forecasting method based on the ARIMA-LSTM combined model, such as figure 1 , A short-term building load forecasting method based on the ARIMA-LSTM combined model includes collecting influencing factor data through a data collector, and performing maximum and minimum normalization processing on the load data and each influencing factor data to obtain a dimensionless data set;
[0066] Select key influencing factors;
[0067] Calculate the cosine similarity and obtain the sample data of similar days as the training set;
[0068] Input the similar daily load training set into the ARIMA-LSTM combined model to obtain the load forecast result;
[0069] Wherein, the influencing factor data includes load data, weather data and date type data.
[0070] Specifically, the main body of the present invention includes S1: Collect influencing factor data through a data coll...
Embodiment 2
[0110] This embodiment is to verify and explain the technical effects used in the method. The different methods selected in this embodiment and the method are used for comparative testing, and the test results are compared by means of scientific demonstration to verify the true effects of the method.
[0111] First, collect the data of building load influencing factors through the data collector, including load data, meteorological data and date type data. The data sampling interval is 1 hour, and 24 data points are recorded every day; then the analysis and screening of building load forecasting When training sample data, analyze the load influencing factors, then preprocess the original sequence data, and finally select similar daily sequence data according to the gray correlation degree and cosine distance.
[0112] Among them, such as figure 1 As shown, the analysis and operation steps of the collected data are described as follows:
[0113] 1) Since meteorological factors have a...
PUM
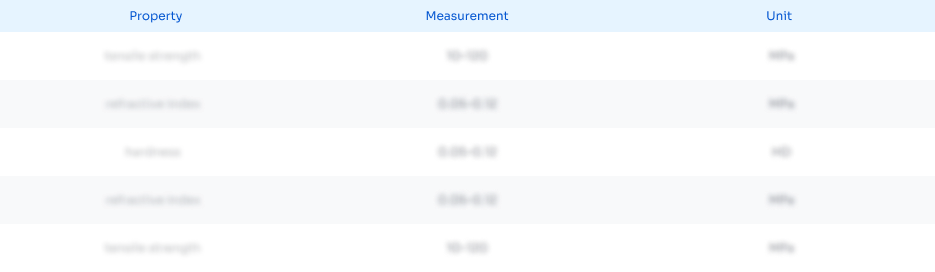
Abstract
Description
Claims
Application Information
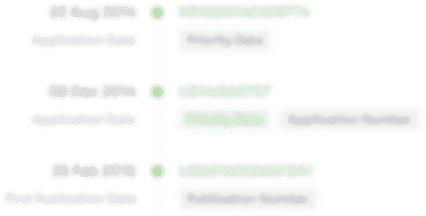
- R&D Engineer
- R&D Manager
- IP Professional
- Industry Leading Data Capabilities
- Powerful AI technology
- Patent DNA Extraction
Browse by: Latest US Patents, China's latest patents, Technical Efficacy Thesaurus, Application Domain, Technology Topic, Popular Technical Reports.
© 2024 PatSnap. All rights reserved.Legal|Privacy policy|Modern Slavery Act Transparency Statement|Sitemap|About US| Contact US: help@patsnap.com