Image recognition method based on optimized deep neural network model
A deep neural network and model technology, applied in the field of image recognition, can solve problems such as increased model noise, average gradient decrease of network parameters, and decreased training performance, achieving the effects of speeding up, improving image recognition performance, and improving accuracy
- Summary
- Abstract
- Description
- Claims
- Application Information
AI Technical Summary
Problems solved by technology
Method used
Image
Examples
Embodiment Construction
[0036] Preferred embodiments of the present invention will be described in detail below in conjunction with the accompanying drawings, wherein the accompanying drawings constitute a part of the application and together with the embodiments of the present invention are used to explain the principle of the present invention and are not intended to limit the scope of the present invention.
[0037] A specific embodiment of the present invention, such as figure 1 Shown, disclose a kind of image recognition method based on optimizing deep neural network model, comprise the following steps:
[0038] S1, select the data set of the image as the training sample, divide the data set into multiple sample sets according to the batch setting of the batch number, input the current sample set into a specific deep neural network model, and obtain the loss function value set corresponding to the current sample set ; Wherein, the specific deep neural network model is selected as a deep neural n...
PUM
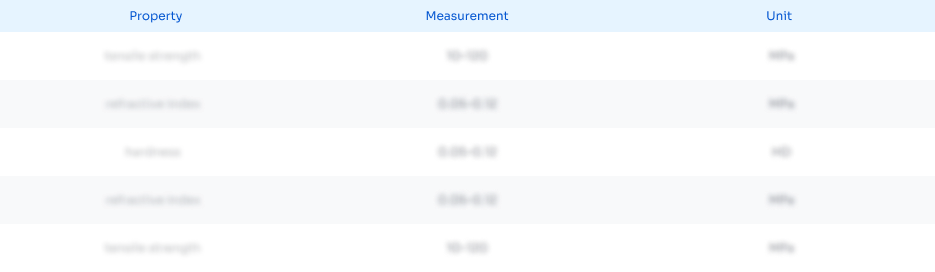
Abstract
Description
Claims
Application Information
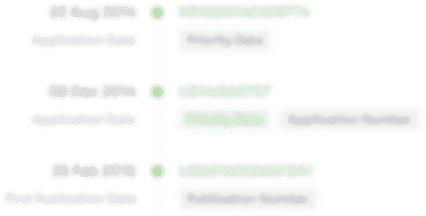
- R&D Engineer
- R&D Manager
- IP Professional
- Industry Leading Data Capabilities
- Powerful AI technology
- Patent DNA Extraction
Browse by: Latest US Patents, China's latest patents, Technical Efficacy Thesaurus, Application Domain, Technology Topic.
© 2024 PatSnap. All rights reserved.Legal|Privacy policy|Modern Slavery Act Transparency Statement|Sitemap