Generative adversarial network embedded representation learning method
A learning method and embedded technology, applied in neural learning methods, biological neural network models, instruments, etc., can solve problems such as poor entity alignment, achieve good entity alignment, simplify parameter settings, and optimize alignment performance.
- Summary
- Abstract
- Description
- Claims
- Application Information
AI Technical Summary
Problems solved by technology
Method used
Image
Examples
Embodiment Construction
[0031] The present invention will be described in detail below in conjunction with the accompanying drawings and embodiments.
[0032] In recent years, generative adversarial networks have been widely used in solving problems with complex data distributions. Generative Adversarial Network (Generative Adversarial Network) is mainly composed of a generative network (Generator, G) and a discriminative network (Discriminator, Discriminator, D): the generator is used to learn from a given noise distribution (usually Uniform distribution or normal distribution), the discriminator needs to distinguish whether the given data is the real data in the data set or the fake data generated by the generator. The generator needs to fit the distribution of real data as much as possible to generate deceptive data, and the discriminator needs to improve its own discrimination ability to prevent being deceived by the fake data of the generator. Throughout the process of the max-min game, the gen...
PUM
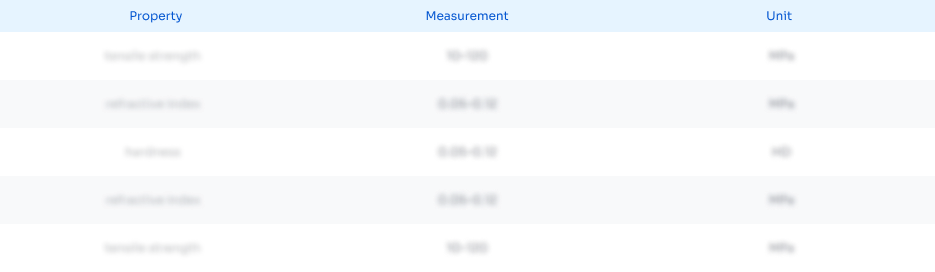
Abstract
Description
Claims
Application Information
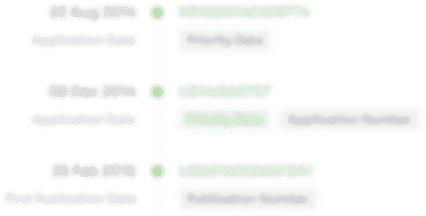
- R&D Engineer
- R&D Manager
- IP Professional
- Industry Leading Data Capabilities
- Powerful AI technology
- Patent DNA Extraction
Browse by: Latest US Patents, China's latest patents, Technical Efficacy Thesaurus, Application Domain, Technology Topic, Popular Technical Reports.
© 2024 PatSnap. All rights reserved.Legal|Privacy policy|Modern Slavery Act Transparency Statement|Sitemap|About US| Contact US: help@patsnap.com