Image segmentation method based on convolutional network
A convolutional network and image segmentation technology, applied in biological neural network models, instruments, character and pattern recognition, etc., can solve the problems of ignoring real-time requirements, too much category information, and insufficiently clear semantic object contours.
- Summary
- Abstract
- Description
- Claims
- Application Information
AI Technical Summary
Problems solved by technology
Method used
Image
Examples
Embodiment approach 1
[0101] Step 1. Image dataset preprocessing.
[0102] Using the Cityscapes image dataset, the dataset contains 5000 pictures, 2975 training sets, 500 validation sets and 1525 test sets, with a resolution size of 1024×2048, subdivided into 34 different segmentation categories. Since some categories account for too little in the entire data set, the measurement index is calculated as 0 when testing the segmentation results, which affects the overall evaluation results. Therefore, only 11 categories are used in the training. Through calculation, the 11 categories The proportion of pixels has exceeded 90% of the total number of pixels, respectively road (Road), sidewalk (Sidewalk), building (Building), vegetation (Vegetation), sky (Sky), terrain (Terrain), person (Person) , Car (Car), Bicycle (Bicycle), Pole (Pole), Bus (Bus). At the same time, the images in the training set were folded left and right to expand the data set, and 5950 images were obtained, and then the image size w...
Embodiment approach 2
[0114] The difference between this embodiment and Embodiment 1 is that in the step 2, a convolutional network is designed and network training is performed. In this embodiment, a multi-resolution strategy is used for network training. First scale the data to three different resolution sizes, full
[0115] The resolution is 512×1024, the half resolution is 256×512, and the three-quarter resolution is 384×768. After that, the half-resolution data set is trained first, and the network parameters are used as the training initialization parameters of the three-quarter resolution data set. Finally train on the full resolution dataset. On the one hand, the data set is indirectly expanded through different resolutions, and at the same time, the same image area is encouraged to use the same label at different resolutions to strengthen the interaction between pixels. Other steps and parameters are the same as those in Embodiment 1.
Embodiment approach 3
[0117] The difference between this embodiment and Embodiments 1 and 2 is that the model optimization and improvement process in step 4, in this embodiment, the optimized network model parameters in step 4 are processed, the BN layer parameters are inferred, and merged into the volume In the stacking layer, the inference speed of the network model is optimized. The BN layer is often used in the training phase of the network. By performing batch normalization operations on the input data, the convergence rate of the network is accelerated and the problems of gradient disappearance and gradient explosion are avoided. The specific method of merging parameters is as follows: assuming that the training weight obtained in a certain convolutional layer of the network is W, and the deviation parameter is b, the convolution operation can be simplified as Y=WX+b, and X is the input of the previous layer of the network. Let the mean in the BN layer be μ, the variance be δ, the scaling fac...
PUM
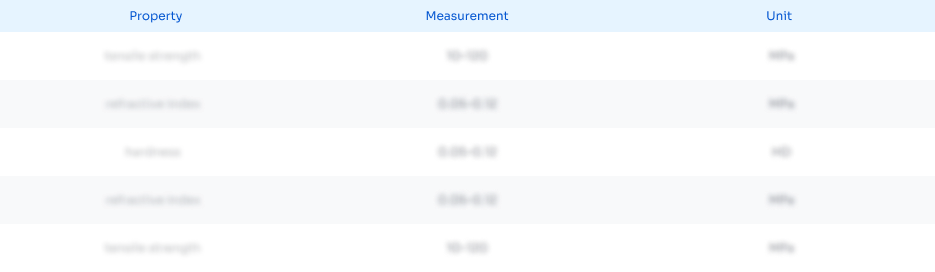
Abstract
Description
Claims
Application Information
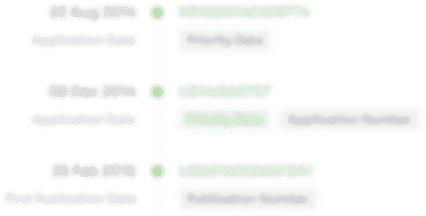
- R&D
- Intellectual Property
- Life Sciences
- Materials
- Tech Scout
- Unparalleled Data Quality
- Higher Quality Content
- 60% Fewer Hallucinations
Browse by: Latest US Patents, China's latest patents, Technical Efficacy Thesaurus, Application Domain, Technology Topic, Popular Technical Reports.
© 2025 PatSnap. All rights reserved.Legal|Privacy policy|Modern Slavery Act Transparency Statement|Sitemap|About US| Contact US: help@patsnap.com