A noise image segmentation method based on improved energy functional model
An energy functional and image segmentation technology, applied in the field of image processing, to achieve the effect of improving segmentation speed and segmentation accuracy, reducing staircase phenomenon, and maintaining integrity
- Summary
- Abstract
- Description
- Claims
- Application Information
AI Technical Summary
Problems solved by technology
Method used
Image
Examples
Embodiment 1
[0066] Such as figure 1 As shown, this embodiment relates to a noise image segmentation method based on an improved energy functional model, which specifically includes the following steps:
[0067] (1) Input the original image I(x, y);
[0068] (2) Using an energy functional model based on a non-convex functional to denoise the original image I (x, y) input in step (1), to obtain a smooth image u after denoising, the energy functional model as follows:
[0069]
[0070] Among them: 00 is the adjustment parameter, is the gradient operator;
[0071] (3) An initialization curve C is given to the smooth image u in step (2), and the initial parameters of the initialization curve C are set as follows:
[0072] The interval of the discrete grid is h=1, the time step is Δt=0.1, the regularization parameter ε=1, the weight of the length penalty item L(C) μ=O*255 2 , where O∈[0,1];
[0073] (4) According to the variational method and the Euler-Lagrange equation, the evolution...
Embodiment 2
[0093] This embodiment verifies the image noise processing capability of the present invention.
[0094] Such as figure 2 Shown in (a), it is three images with high-intensity noise, using the method of the present invention to carry out noise processing and segmentation, by figure 2 (b) It can be seen that the method of the present invention achieves better results in target segmentation under high-intensity noise.
[0095] In order to realize the qualitative evaluation of the method of the present invention, three measures of True Positive Rate (True Positive Rate, TPR), False Positive Rate (False Positive Rate, FPR) and Similarity Index (Similarity Index, SI) are used for evaluation. The definitions of the three metrics are as follows:
[0096]
[0097] Among them, S T Indicates the real foreground pixel set of the image to be segmented in the image, S A Indicates the pixel set of the foreground area obtained by the model segmentation algorithm. Ideally, SI and TPR ...
Embodiment 3
[0102] In this embodiment, the method of the present invention is compared with the image segmentation results of the NLIRCV algorithm. For details of the NLIRCV algorithm, please refer to the literature PIOTR S B, PAPIEZ J A, SCHNABEL C M.A level-set approach to joint image segmentation and registration with application to CT lung imaging [J]. Computerized Medical Imaging and Graphics, 2018, 65: 58-68, this The NLIRCV algorithm referred to in the invention is the abbreviation of Chan-Vese segmentation algorithm with a non-linear intensity-based registration algorithm in the above literature.
[0103] Such as image 3 As shown in (a), it is the original image and the image after adding different degrees of noise to the original image, and the latter two images are images with the standard deviation of 0.01 and 0.02 Gaussian noise added respectively. In the experiment, μ=0.06*255 of the two algorithms 2 .
[0104] Adopt the method of the present invention and NLIRCV algorith...
PUM
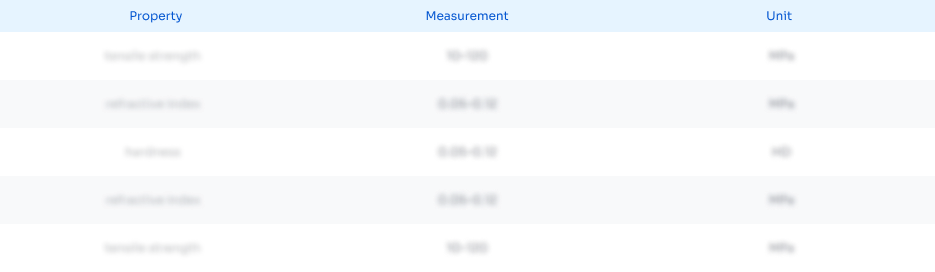
Abstract
Description
Claims
Application Information
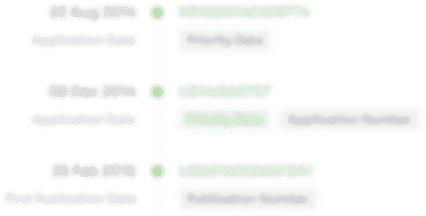
- R&D
- Intellectual Property
- Life Sciences
- Materials
- Tech Scout
- Unparalleled Data Quality
- Higher Quality Content
- 60% Fewer Hallucinations
Browse by: Latest US Patents, China's latest patents, Technical Efficacy Thesaurus, Application Domain, Technology Topic, Popular Technical Reports.
© 2025 PatSnap. All rights reserved.Legal|Privacy policy|Modern Slavery Act Transparency Statement|Sitemap|About US| Contact US: help@patsnap.com