Double-flow network behavior recognition method based on multi-level spatial-temporal feature fusion enhancement
A technology of spatiotemporal features and recognition methods, applied in character and pattern recognition, biological neural network models, instruments, etc., can solve problems such as weakening effects, and achieve the effect of improving the accuracy of behavior recognition
- Summary
- Abstract
- Description
- Claims
- Application Information
AI Technical Summary
Problems solved by technology
Method used
Image
Examples
Embodiment Construction
[0027] figure 2 It is an overall model diagram of the present invention;
[0028] figure 2 It represents the algorithm model diagram of the present invention. The algorithm takes multi-segment RGB images and optical flow maps as input, and the model includes five key parts: spatial network, temporal network, feature fusion network, multi-segment category probability distribution fusion and multi-flow category probability distribution fusion. Both the spatial network and the temporal network are built based on InceptionV3, and the feature fusion network is constructed through the spatial network and the temporal network. Simply put, the proposed multi-level spatiotemporal feature fusion module is used to fuse spatiotemporal mixed features of different depth levels, where the spatiotemporal mix The feature is to use the proposed spatio-temporal feature fusion module to fuse the features extracted from the spatial network and the temporal network, and then use the proposed gr...
PUM
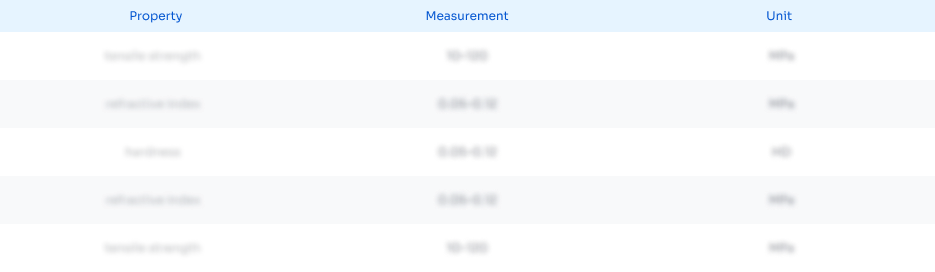
Abstract
Description
Claims
Application Information
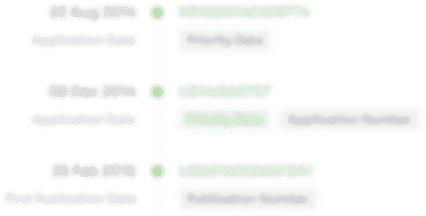
- R&D Engineer
- R&D Manager
- IP Professional
- Industry Leading Data Capabilities
- Powerful AI technology
- Patent DNA Extraction
Browse by: Latest US Patents, China's latest patents, Technical Efficacy Thesaurus, Application Domain, Technology Topic.
© 2024 PatSnap. All rights reserved.Legal|Privacy policy|Modern Slavery Act Transparency Statement|Sitemap