Quantum generative adversarial network algorithm based on conditional constraints
A quantum and conditional technology, applied in the field of quantum generative adversarial network algorithms based on conditional constraints, which can solve problems such as difficult to control data
- Summary
- Abstract
- Description
- Claims
- Application Information
AI Technical Summary
Problems solved by technology
Method used
Image
Examples
Embodiment Construction
[0039] The present invention will be further described in detail below in conjunction with specific implementation methods and accompanying drawings.
[0040] Such as figure 1 As shown, the quantum generation confrontation network algorithm based on conditional constraints of the present invention comprises the following steps:
[0041] (1) Prepare real samples, record the sample data set as X={x 1 ,x 2 ,...,x n}∈R, and the data set conforms to an unknown probability distribution ρ data . Introduce appropriate conditional constraints according to the goal of the generation task and the numerical characteristics of the sample data, denoted as y={y 1 ,y 2 ,...,y m}; then the sample data and condition variables together constitute the training data set of the Generative Adversarial Network, denoted as {(x 1 ,c 1 ),…,(x i ,c i ),…,(x n ,c n )}(x i ∈X,c i ∈y);
[0042] (2) Quantum generation model preparation: prepare the data pairs in the training data set into qua...
PUM
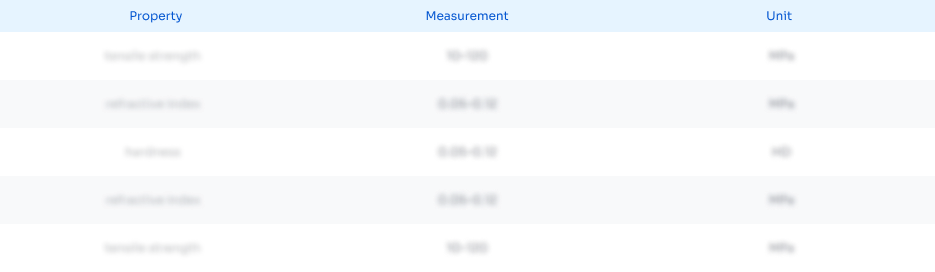
Abstract
Description
Claims
Application Information
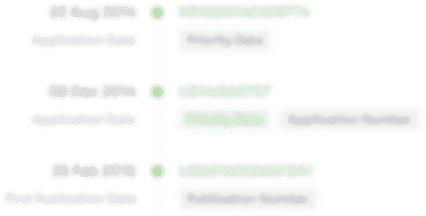
- Generate Ideas
- Intellectual Property
- Life Sciences
- Materials
- Tech Scout
- Unparalleled Data Quality
- Higher Quality Content
- 60% Fewer Hallucinations
Browse by: Latest US Patents, China's latest patents, Technical Efficacy Thesaurus, Application Domain, Technology Topic, Popular Technical Reports.
© 2025 PatSnap. All rights reserved.Legal|Privacy policy|Modern Slavery Act Transparency Statement|Sitemap|About US| Contact US: help@patsnap.com