Wind power prediction method and system integrating long-term and short-term memory network and extreme learning machine
A technology of extreme learning machine and long-term short-term memory, applied in neural learning methods, prediction, biological neural network models, etc., can solve problems such as poor prediction effect
- Summary
- Abstract
- Description
- Claims
- Application Information
AI Technical Summary
Problems solved by technology
Method used
Image
Examples
Embodiment 1
[0031] In the technical solutions disclosed in one or more embodiments, such as figure 1 As shown, the wind power prediction method that integrates long-term short-term memory network and extreme learning machine includes the following steps:
[0032] Step 1. Obtain the wind power sequence and corresponding meteorological data, respectively preprocess the wind power sequence and meteorological characteristic data, and obtain the characteristic data and meteorological characteristic data of the wind power sequence;
[0033] Step 2. Recombine the feature data of the wind power sequence and the meteorological feature data according to the frequency to form a low-frequency combined input feature vector and a high-frequency combined input feature vector:
[0034] Specifically, the low-frequency component of the wind power sequence and the principal component information affecting wind power generation in the meteorological data can be combined as the first feature vector, which is ...
Embodiment 2
[0119] This embodiment provides a wind power forecasting system that integrates long-term short-term memory networks and extreme learning machines, such as image 3 shown, including:
[0120] Acquisition and preprocessing module: configured to obtain wind power sequence and corresponding meteorological data, perform preprocessing to obtain characteristic data and meteorological characteristic data of wind power sequence;
[0121] Classification module: configured to recombine the feature data and meteorological feature data of the wind power sequence according to the frequency to form a low-frequency combined input feature vector and a high-frequency combined input feature vector;
[0122] Prediction module: configured to input the low-frequency combination input feature vector to the trained long-term short-term memory network prediction model to obtain the first prediction result, and input the high-frequency combination input feature vector to the trained extreme learning m...
Embodiment 3
[0125] This embodiment provides an electronic device, including a memory, a processor, and computer instructions stored in the memory and executed on the processor. When the computer instructions are executed by the processor, the steps described in the method in Embodiment 1 are completed.
PUM
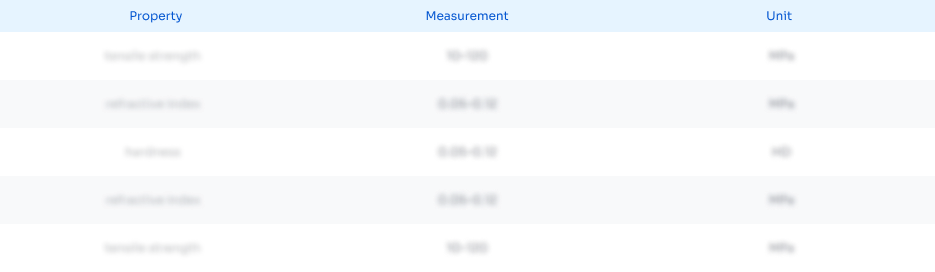
Abstract
Description
Claims
Application Information
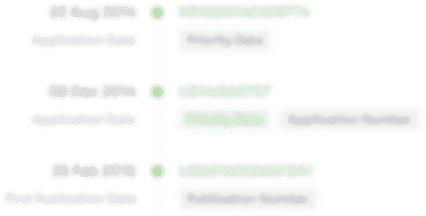
- R&D
- Intellectual Property
- Life Sciences
- Materials
- Tech Scout
- Unparalleled Data Quality
- Higher Quality Content
- 60% Fewer Hallucinations
Browse by: Latest US Patents, China's latest patents, Technical Efficacy Thesaurus, Application Domain, Technology Topic, Popular Technical Reports.
© 2025 PatSnap. All rights reserved.Legal|Privacy policy|Modern Slavery Act Transparency Statement|Sitemap|About US| Contact US: help@patsnap.com