Dense crowd counting method of multi-scale convolutional neural network
A technology of convolutional neural network and dense crowd, applied in the field of dense crowd counting of multi-scale convolutional neural network, can solve the problems of gradient disappearance, poor feature extraction, over-fitting of deep convolutional neural network, etc. Effects of denaturation, guaranteed scale invariance, and low hardware requirements
- Summary
- Abstract
- Description
- Claims
- Application Information
AI Technical Summary
Problems solved by technology
Method used
Image
Examples
Embodiment 1
[0052] Embodiment 1: to the inspection of mainstream data set Shanghai Tech Part_A data set;
[0053] Shanghai Tech dataset: The Shanghai Tech dataset consists of 1198 pictures, of which 482 pictures belong to Shanghai Tech Part_A, and 716 pictures belong to Shanghai Tech Part_B. Part_A and Part_B are two independent parts, such as Figure 4 shown;
[0054] Among them, Shanghai Tech Part_A has a relatively dense crowd, and includes indoor and outdoor crowd pictures, including color pictures and black and white pictures;
[0055] The training set and test set of Part_A are 300 pictures and 182 pictures respectively. Among these pictures, the number of people is 3139, and the number of people is 33, such as Figure 5 As shown, in the training set of Part_A, there are 230 pictures with the largest number of people under 700 people, and only 1 picture with the least number of people with more than 2800 people and less than 3500 people.
[0056] In Part_A, the network model of th...
Embodiment 2
[0057] Example 2: Inspection of the mainstream data set Shanghai Tech Part_B data set
[0058] Shanghai Tech dataset: The Shanghai Tech dataset consists of 1198 pictures, of which 482 pictures belong to Shanghai Tech Part_A, and 716 pictures belong to Shanghai Tech Part_B. Part_A and Part_B are two independent parts, such as Figure 4 shown;
[0059] The Shanghai Tech dataset Part_B has 716 pictures, all of which were taken from above on the streets of Shanghai, including black and white and color pictures. The training set and test set of Part_B are 400 pictures and 316 pictures respectively. Such as Image 6 As shown, in the training set of Part_B, there are 213 pictures with the largest number of people under 100; the least pictures with more than 400 people and less than 500 people are only 7 pictures; in the test set of Part_B, the pictures with the number of people less than 100 The most, there are 169 pictures; the pictures with more than 500 people and less than 600...
Embodiment 3
[0061] Example 3: Inspection of the mainstream data set UCF_CC_50 data set
[0062] The UCF_CC_50 data set has only 50 black and white pictures in total, and there is no training set and test set, such as Figure 4 shown.
[0063] The number of people in these pictures has a large span, the largest number is 4543 people, and the smallest number is 94 people. The distribution of the number of people in the data set pictures is as follows Figure 7 As shown, there are 25 pictures with the number of people less than 1000 the most; pictures with more than 3000 people and less than 4000 people are the least, only 2 pictures.
[0064] On the UCF_CC_50 dataset, the network model of the SPMsCNN algorithm takes about 1.34 seconds to run a picture. Such as Figure 9 As shown, the SPMsCNN algorithm achieved the best experimental results on the UCF_CC_50 dataset. Compared with the ADR (ADeeply-Recursive, ADR) algorithm, the MAE and MSE of the SPMsCNN algorithm were reduced by 36.8 and ...
PUM
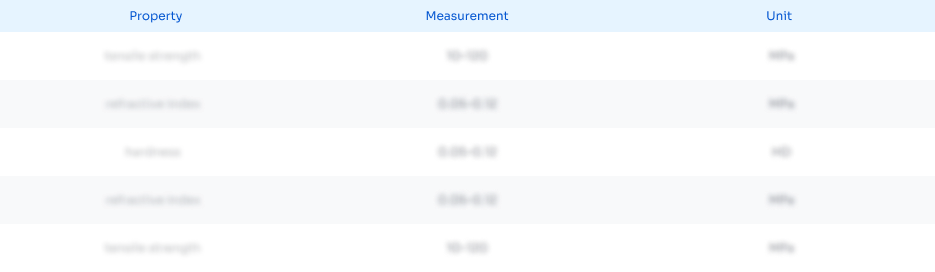
Abstract
Description
Claims
Application Information
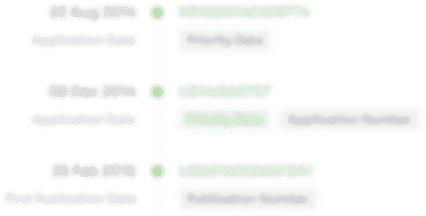
- R&D Engineer
- R&D Manager
- IP Professional
- Industry Leading Data Capabilities
- Powerful AI technology
- Patent DNA Extraction
Browse by: Latest US Patents, China's latest patents, Technical Efficacy Thesaurus, Application Domain, Technology Topic, Popular Technical Reports.
© 2024 PatSnap. All rights reserved.Legal|Privacy policy|Modern Slavery Act Transparency Statement|Sitemap|About US| Contact US: help@patsnap.com