Multi-scale self-attention target detection method based on weight sharing
A technology of weight sharing and target detection, which is applied in the field of computer vision, can solve problems such as disappearance and gradient dispersion, and achieve the effect of reducing time consumption
- Summary
- Abstract
- Description
- Claims
- Application Information
AI Technical Summary
Problems solved by technology
Method used
Image
Examples
Embodiment Construction
[0041] The present invention will be further described in detail below in conjunction with the accompanying drawings and specific embodiments. However, it should not be interpreted that the scope of the above-mentioned theme of the present invention is limited to the following embodiments, and all technologies realized based on the contents of the present invention belong to the scope of this aspect.
[0042] The overall implementation process of a multi-scale self-attention target detection model based on weight sharing provided by the present invention is as follows: figure 1 As shown, the specific description is as follows:
[0043] The training set in PASCALVOC2012 is selected as the training data. The present invention removes pictures that are too large or too small in some pictures, and screens out label data for target detection. A total of 3,000 training images of different backgrounds and different target categories, 500 verification images, and 500 test samples we...
PUM
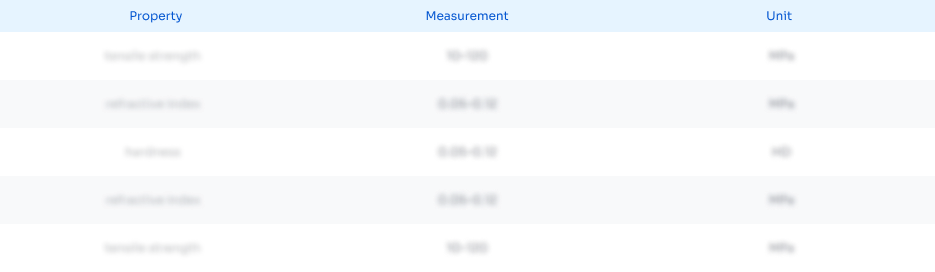
Abstract
Description
Claims
Application Information
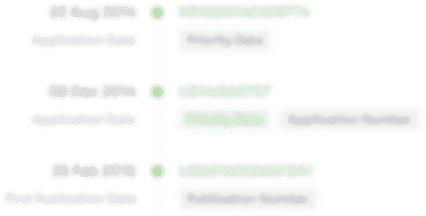
- R&D Engineer
- R&D Manager
- IP Professional
- Industry Leading Data Capabilities
- Powerful AI technology
- Patent DNA Extraction
Browse by: Latest US Patents, China's latest patents, Technical Efficacy Thesaurus, Application Domain, Technology Topic, Popular Technical Reports.
© 2024 PatSnap. All rights reserved.Legal|Privacy policy|Modern Slavery Act Transparency Statement|Sitemap|About US| Contact US: help@patsnap.com