Liver pathological image segmentation model establishment and segmentation method based on attention mechanism
A technology of pathological image and segmentation model, applied in the field of medical image analysis, can solve the problem of inaccurate boundary segmentation between normal tissue area and abnormal tissue area
- Summary
- Abstract
- Description
- Claims
- Application Information
AI Technical Summary
Problems solved by technology
Method used
Image
Examples
Embodiment 1
[0084] This embodiment discloses a method for establishing a liver pathological image segmentation model based on an attention mechanism, which specifically includes the following steps:
[0085] Step 1, liver tissue pathological image processing
[0086] The data set in this example is derived from 30 full-field pathological slices of H&E stained liver histopathology collected by the pathology department of a hospital in China. The data set is randomly divided into three parts, and three-fold cross-validation is used to evaluate the network. The folded mean is used as the final result.
[0087] The image processed by the present invention is a digital pathological full-field image (RGB, 3 channels), and the image size is more than 10000╳10000. Due to the limitation of computer performance, it is generally impossible to directly segment the full-field pathological image, so the sliding window strategy is used. Crop the full-field image into 512×512 image blocks. However, in ...
Embodiment 2
[0124] This embodiment discloses a system for establishing a liver pathological image segmentation model based on an attention mechanism, such as Figure 5 As shown, the system includes:
[0125] (1) The image processing module is used for cropping the liver tissue pathological slice image and its corresponding expert annotated mask image to obtain slice image blocks and mask image blocks; it specifically includes an image cropping module and an image preprocessing module, wherein ,
[0126] (1.1) Image cropping module, which is used for cropping the liver tissue pathological slice image and its corresponding expert annotated mask image,
[0127] (1.2) Image preprocessing module, which is used to uniformly map the background of sliced image blocks and mask image blocks to [255, 255, 255] by setting a threshold, and remove the black area generated by the sliding window strategy clipping to the boundary.
[0128] (2) Segmentation network building module, which is used to con...
Embodiment 3
[0154] This embodiment discloses a liver pathological image segmentation method based on an attention mechanism, which includes the following steps:
[0155] Step 1, the liver pathological image to be processed.
[0156] In this embodiment, 38 groups of 197 groups of liver histopathological image blocks are used as test sets, such as Image 6 The central image shows one of the 38 groups of image blocks. Process according to step 1 in Embodiment 1 to obtain slice image blocks and mask image blocks;
[0157] Step 2, input the obtained slice image block and mask image block obtained in step 1 into the segmentation model obtained in claim 1 to obtain a segmentation probability map, wherein the segmentation probability map includes a target segmentation probability map and a background segmentation probability map; compare The size of the probability value of each pixel on the target segmentation probability map and the background segmentation probability map, and its label is th...
PUM
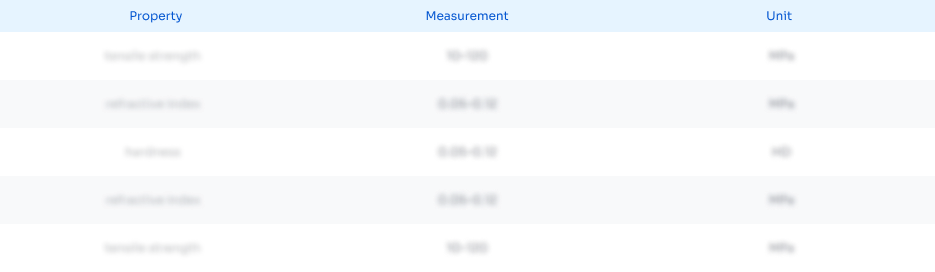
Abstract
Description
Claims
Application Information
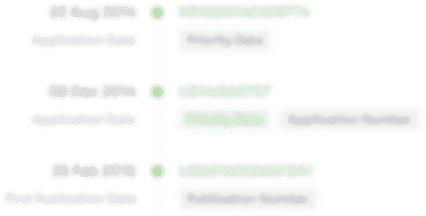
- R&D Engineer
- R&D Manager
- IP Professional
- Industry Leading Data Capabilities
- Powerful AI technology
- Patent DNA Extraction
Browse by: Latest US Patents, China's latest patents, Technical Efficacy Thesaurus, Application Domain, Technology Topic, Popular Technical Reports.
© 2024 PatSnap. All rights reserved.Legal|Privacy policy|Modern Slavery Act Transparency Statement|Sitemap|About US| Contact US: help@patsnap.com