Hyperspectral image denoising method based on non-convex low-rank matrix approximation and total variation regularization
A technology of hyperspectral image and low-rank matrix, applied in the field of hyperspectral image denoising, it can solve the problems of large singular value penalty and inability to treat different singular values equally, and achieve the effect of maintaining edge information and improving smoothness.
- Summary
- Abstract
- Description
- Claims
- Application Information
AI Technical Summary
Problems solved by technology
Method used
Image
Examples
Embodiment Construction
[0048] The technical solution of the present invention will be further described below in conjunction with the accompanying drawings.
[0049] The method of combining non-convex low-rank matrix approximation and full variation regularization to remove mixed noise includes the following steps:
[0050] Step 1) Input a hyperspectral image contaminated by Gaussian noise, impulse noise, etc. Among them, M, N, and K respectively represent the width, height, and number of bands of the hyperspectral image, specifically as figure 1 shown;
[0051] Step 2) The denoising model based on non-convex low-rank matrix factorization and TV regularization is defined as follows:
[0052]
[0053] in Φ represents the reconstruction of the vector of the K-th band into a two-dimensional matrix of M×N. S represents the sparse error matrix, ||·|| F Represents the Frobenius norm. δ is a constant, ρ, λ are balance parameters;
[0054] Step 3) adopting the augmented Lagrange function algo...
PUM
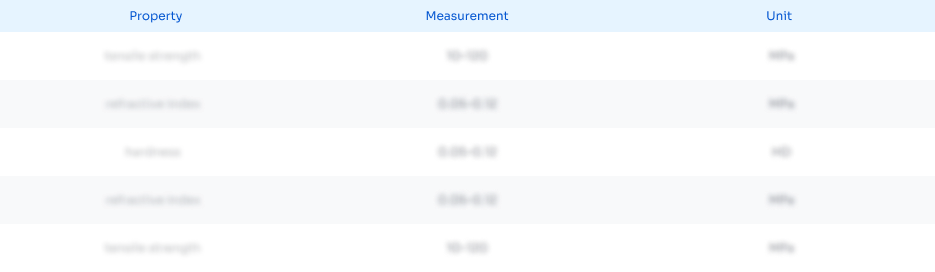
Abstract
Description
Claims
Application Information
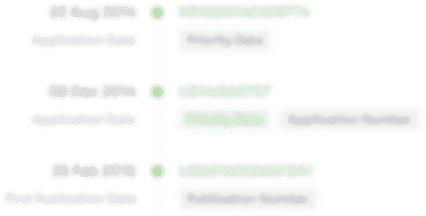
- R&D Engineer
- R&D Manager
- IP Professional
- Industry Leading Data Capabilities
- Powerful AI technology
- Patent DNA Extraction
Browse by: Latest US Patents, China's latest patents, Technical Efficacy Thesaurus, Application Domain, Technology Topic, Popular Technical Reports.
© 2024 PatSnap. All rights reserved.Legal|Privacy policy|Modern Slavery Act Transparency Statement|Sitemap|About US| Contact US: help@patsnap.com