Heterogeneous graph classification method based on double-layer attention mechanism
A classification method and attention technology, applied in neural learning methods, computer components, instruments, etc., can solve problems such as information loss, exhaustion of multiple meta-paths of semantic information, and failure to consider node type information, etc., to reduce information loss , the effect of improving accuracy
- Summary
- Abstract
- Description
- Claims
- Application Information
AI Technical Summary
Problems solved by technology
Method used
Image
Examples
Embodiment 1
[0048] This embodiment provides a heterogeneous graph classification method based on a two-layer attention mechanism, such as figure 1 shown, including steps:
[0049] S11. Mapping the eigenvectors of different types of nodes to obtain the eigenvectors of the target node and the neighborhood nodes corresponding to the target node in the same entity space;
[0050] S12. According to the obtained feature vectors, and based on the type-level attention and node-level attention in the double-layer attention, from the type-level attention to the node-level attention, learn from top to bottom between different types of neighbors and different adjacent nodes. the weight of;
[0051] S13. Construct a heterogeneous graph according to the obtained weights to obtain a classification model;
[0052] S14. Input the test data into the obtained classification model, and output the final classification result.
[0053] The specific idea of this embodiment: the present invention uses a two...
PUM
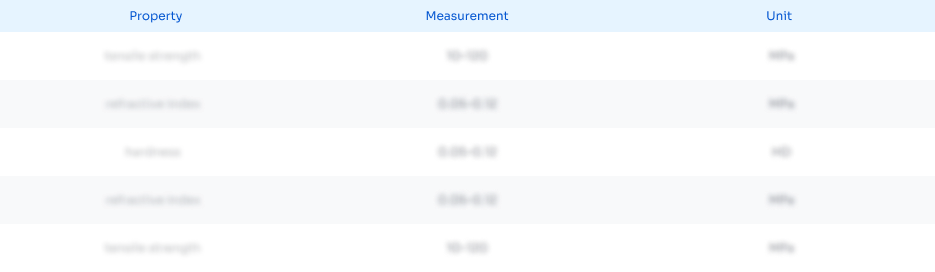
Abstract
Description
Claims
Application Information
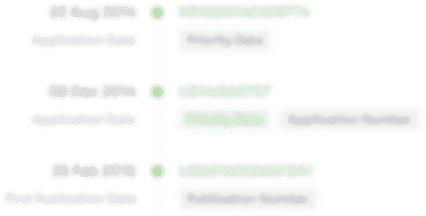
- R&D Engineer
- R&D Manager
- IP Professional
- Industry Leading Data Capabilities
- Powerful AI technology
- Patent DNA Extraction
Browse by: Latest US Patents, China's latest patents, Technical Efficacy Thesaurus, Application Domain, Technology Topic, Popular Technical Reports.
© 2024 PatSnap. All rights reserved.Legal|Privacy policy|Modern Slavery Act Transparency Statement|Sitemap|About US| Contact US: help@patsnap.com