Multi-view clustering method based on constrained non-negative matrix factorization
A technology of non-negative matrix decomposition and clustering method, which is applied in the field of multi-view clustering based on constrained non-negative matrix decomposition, and can solve problems such as poor performance of multivariate time series data
- Summary
- Abstract
- Description
- Claims
- Application Information
AI Technical Summary
Problems solved by technology
Method used
Image
Examples
Embodiment Construction
[0070] The technical solution of the present invention will be described in detail below in conjunction with the drawings and embodiments.
[0071] Aiming at the clustering problem of high-dimensional multivariate time series, the present invention proposes a multi-view clustering method based on constrained non-negative matrix decomposition, and simultaneously considers the consistency between different views and the particularity information of each view to realize the multivariate time series Clustering of data with high accuracy.
[0072] The embodiment of the present invention takes the Auslan data set as a specific example. The Auslan data contains 95 categories, and each data includes 22 variables, that is, each data includes a time series of 3 variables, and the length of the time series in each data is 45 to 316 ranging, including a total of 1140 data. The number of neighbors in the multi-relational network is 7, the number of generated multi-views is 2, and the matr...
PUM
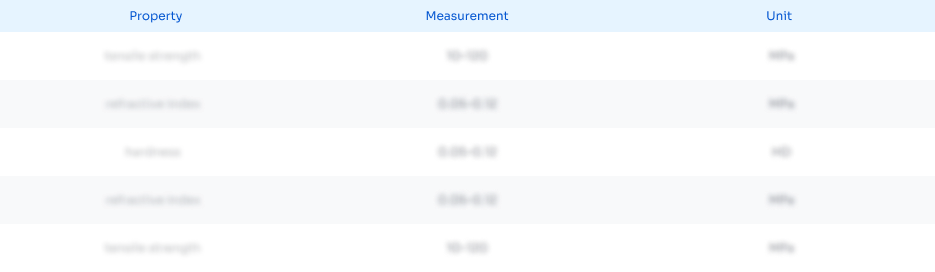
Abstract
Description
Claims
Application Information
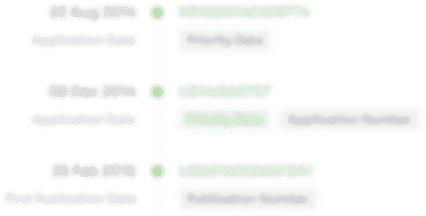
- R&D Engineer
- R&D Manager
- IP Professional
- Industry Leading Data Capabilities
- Powerful AI technology
- Patent DNA Extraction
Browse by: Latest US Patents, China's latest patents, Technical Efficacy Thesaurus, Application Domain, Technology Topic, Popular Technical Reports.
© 2024 PatSnap. All rights reserved.Legal|Privacy policy|Modern Slavery Act Transparency Statement|Sitemap|About US| Contact US: help@patsnap.com