Multivariable input and multivariable output time series prediction method and system
A technology for outputting time and sequence prediction, applied in prediction, instrument, biological neural network model, etc., can solve the problem of inability to take into account the covariance of long-term dependencies and related factors at the same time, and achieve the effect of improving perception ability and prediction accuracy.
- Summary
- Abstract
- Description
- Claims
- Application Information
AI Technical Summary
Problems solved by technology
Method used
Image
Examples
Embodiment 1
[0052] see figure 1 , this embodiment provides a multivariate input and multivariate output time series forecasting method, the specific steps are as follows:
[0053] S1. Prepare multivariate time series data X; multivariate time series data X includes at least one time variable data g t and at least one non-time variable data y t and in chronological order, i.e. x t ={g t ,y t}, multivariate time series data with X t ={x 1 , x 2 ,...,x t}, where t represents the last moment of the sequence, and the data at each moment is n-dimensional data, that is, there are n variables. such as x t ={xx 1 , xx 2 ,...,xx n} t . x t Among the n variables of , there are several variables that only change with time, such as year, month, day information, whether there are holidays, etc. These variables are recorded as g t , and the remaining variables are recorded as y as non-time variables t ,y t The dimension of is denoted as m.
[0054] Examples of multivariate time-series...
Embodiment 2
[0102] see figure 2 , realizing the multivariate input and multivariate output time series prediction system described in Embodiment 1, including
[0103] Convolutional network for extracting time series trend data R of multivariate time series data X;
[0104] The long-period encoding and decoding module includes a long-period encoder and a long-period decoder, wherein the long-period encoder is a self-attention layer, and the long-period decoder includes a self-attention layer and a self-attention decoding layer. Perform correlation calculation on the time series trend data R to obtain the time series trend correlation vector Corr and variable co-correlation vector Corr D ;
[0105] Regression prediction module, used for correlation vector Corr, time series trend data R of historical data and co-correlation vector Corr D Calculate the estimated value of the forecast time series variable Y;
[0106] The model weight training module is used to compare the system output va...
PUM
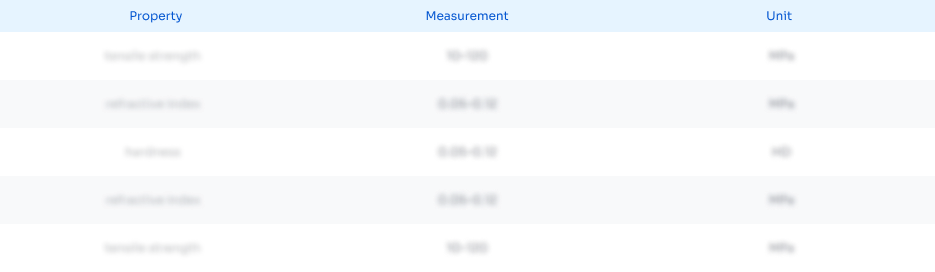
Abstract
Description
Claims
Application Information
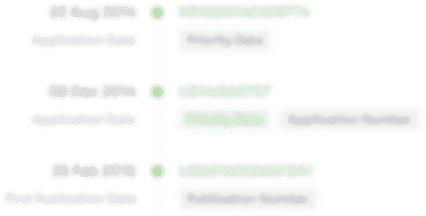
- R&D Engineer
- R&D Manager
- IP Professional
- Industry Leading Data Capabilities
- Powerful AI technology
- Patent DNA Extraction
Browse by: Latest US Patents, China's latest patents, Technical Efficacy Thesaurus, Application Domain, Technology Topic.
© 2024 PatSnap. All rights reserved.Legal|Privacy policy|Modern Slavery Act Transparency Statement|Sitemap