Method, device, equipment and storage medium for constructing forecast model of complex industrial system based on deep learning
A technology for predicting models and industrial systems, applied in neural learning methods, chemical statistics, chemical machine learning, etc., can solve problems such as unknown model structure, high consumption, unknown order of input and output variables, complex dynamic systems, etc. Effects of Die Puzzles
- Summary
- Abstract
- Description
- Claims
- Application Information
AI Technical Summary
Problems solved by technology
Method used
Image
Examples
Embodiment Construction
[0028] The following will clearly and completely describe the technical solutions in the embodiments of the present invention with reference to the accompanying drawings in the embodiments of the present invention. Obviously, the described embodiments are only some, not all, embodiments of the present invention. Based on the embodiments of the present invention, all other embodiments obtained by persons of ordinary skill in the art without creative efforts fall within the protection scope of the present invention.
[0029] figure 1 Realize the flow chart for the complex industrial system forecast model construction method of the embodiment of the present invention, the method includes the following steps:
[0030] S1: Establish a dynamic model of the industrial system, determine the input variables and output variables of the dynamic model, and the output variables are variables to be predicted.
[0031] Specifically, the variable to be predicted is the output variable of the...
PUM
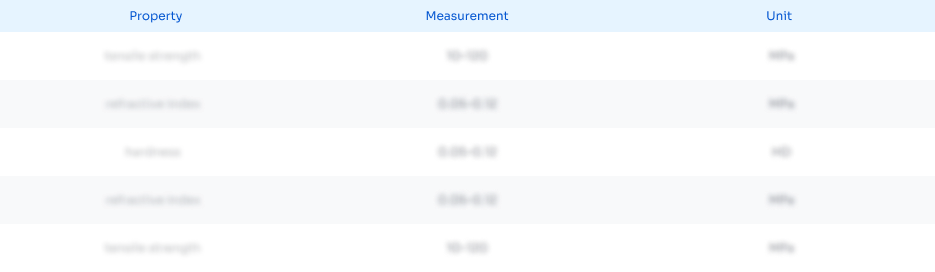
Abstract
Description
Claims
Application Information
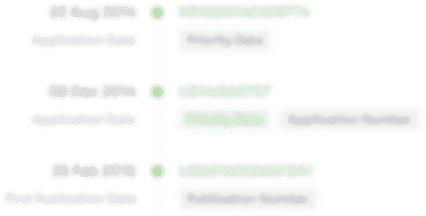
- R&D
- Intellectual Property
- Life Sciences
- Materials
- Tech Scout
- Unparalleled Data Quality
- Higher Quality Content
- 60% Fewer Hallucinations
Browse by: Latest US Patents, China's latest patents, Technical Efficacy Thesaurus, Application Domain, Technology Topic, Popular Technical Reports.
© 2025 PatSnap. All rights reserved.Legal|Privacy policy|Modern Slavery Act Transparency Statement|Sitemap|About US| Contact US: help@patsnap.com