Gait data set synthesis method oriented to complex scene fine-grained attribute driving
A complex scene and data collection technology, applied in the field of computer vision, can solve problems such as difficulty in collecting data, large differences, and low quality of data sets
- Summary
- Abstract
- Description
- Claims
- Application Information
AI Technical Summary
Problems solved by technology
Method used
Image
Examples
preparation example Construction
[0040] refer to figure 1 and figure 2 , in a preferred embodiment of the present invention, a fine-grained attribute-driven gait data set synthesis method for complex scenes is provided, including the following steps:
[0041] S1. Generate several multi-attribute pedestrian 3D models.
[0042] In this embodiment, the specific implementation method of step S1 is:
[0043]Use the MakeHuman tool to randomly generate N 3D character models, each character model has several random character attributes; all 3D character models form a pedestrian 3D model group where M i is the i-th multi-attribute pedestrian 3D model, and N is the total number of models, which is set according to the actual situation.
[0044] Wherein, the random character attributes may include gender, age, weight, ethnicity, etc., and may also include other character attributes, such as height, body shape, and the like.
[0045] S2. Obtain several groups of pedestrian gait movements for binding.
[0046] In ...
Embodiment
[0064] The implementation method of this embodiment is as described above, and the specific steps will not be described in detail. The following only shows the effect of the case data.
[0065] In this embodiment, based on the fine-grained attribute-driven gait data set for complex scenes established by S1-S5, the effect of the generated virtual gait data set is tested by pre-training with the mainstream gait recognition framework. The present invention is tested on two data sets with true value labels, that is, the virtual gait data set D generated by this method is used as a pre-training data set, and the CASIA-B data set is used as a test data set. In the GaitSet and GaitPart methods verified on . see Figure 4 As shown, the data set is as follows:
[0066] Virtual data set: the virtual data set generated by the method of the present invention includes 11268 IDs, 33 perspectives, and 1031844 gait sequences. The data comparison of other mainstream gait data sets in this da...
PUM
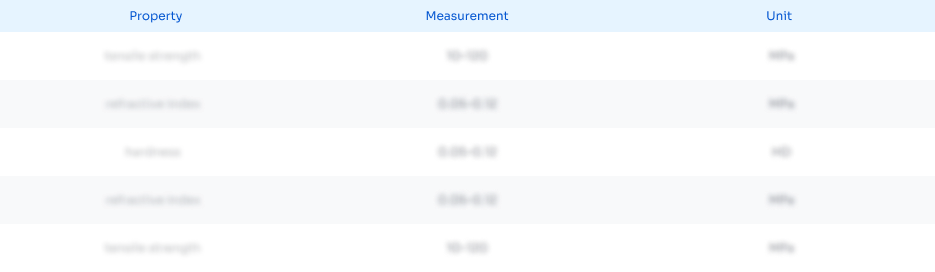
Abstract
Description
Claims
Application Information
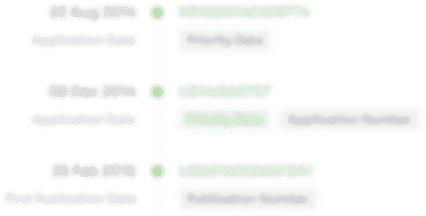
- R&D
- Intellectual Property
- Life Sciences
- Materials
- Tech Scout
- Unparalleled Data Quality
- Higher Quality Content
- 60% Fewer Hallucinations
Browse by: Latest US Patents, China's latest patents, Technical Efficacy Thesaurus, Application Domain, Technology Topic, Popular Technical Reports.
© 2025 PatSnap. All rights reserved.Legal|Privacy policy|Modern Slavery Act Transparency Statement|Sitemap|About US| Contact US: help@patsnap.com