Intelligent fault diagnosis method based on asymmetric domain adversarial self-adaptive model
An adaptive model and fault diagnosis technology, applied in inference methods, neural learning methods, biological neural network models, etc.
- Summary
- Abstract
- Description
- Claims
- Application Information
AI Technical Summary
Problems solved by technology
Method used
Image
Examples
Embodiment Construction
[0047] The present invention will be further described below in conjunction with the accompanying drawings and specific embodiments.
[0048] refer to figure 1 The present invention provides a method for diagnosing faults under variable operating conditions with an asymmetric domain confrontation model, which overcomes the problems of difficulty in adaptive confrontation training in unsupervised domains and large demand for unlabeled data in the target domain. First, the vibration signal collected by the sensor is converted into a time-frequency spectrum, and the domain-invariant fault features are obtained from the time-frequency spectrum by using step-by-step domain confrontation training. At the same time, the lightweight network architecture and Wasserstein distance are integrated into the adversarial network, which greatly reduces the training cost of the network model and reduces the dependence on the target domain data, so that the adversarial migration model still main...
PUM
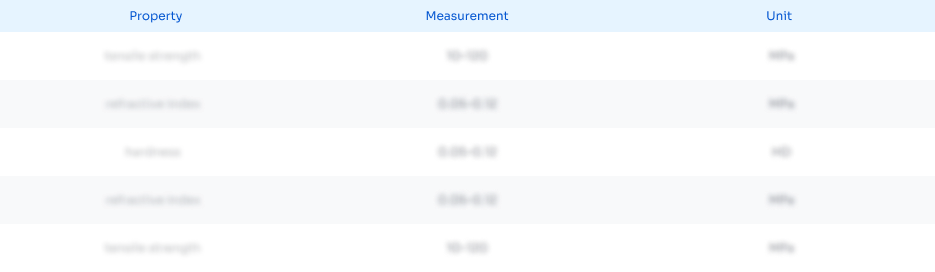
Abstract
Description
Claims
Application Information
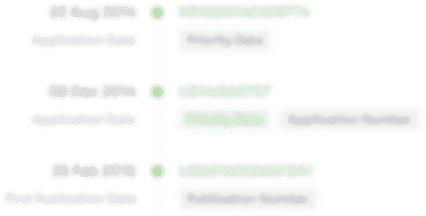
- Generate Ideas
- Intellectual Property
- Life Sciences
- Materials
- Tech Scout
- Unparalleled Data Quality
- Higher Quality Content
- 60% Fewer Hallucinations
Browse by: Latest US Patents, China's latest patents, Technical Efficacy Thesaurus, Application Domain, Technology Topic, Popular Technical Reports.
© 2025 PatSnap. All rights reserved.Legal|Privacy policy|Modern Slavery Act Transparency Statement|Sitemap|About US| Contact US: help@patsnap.com