Meta-learner-based training data generation method and heterogeneous response difference estimation method for causal effects
A technique for training data, learners, applied in the field of machine learning
- Summary
- Abstract
- Description
- Claims
- Application Information
AI Technical Summary
Problems solved by technology
Method used
Image
Examples
Embodiment 1
[0065] Based on the above principles and random field test data, the method for generating training data based on meta-learners provided in this embodiment includes the following steps:
[0066] A1 Obtain two sets of original training data through random field experiments, one set of data is the original training data of the treatment group, and the other set of data is the original training data of the control group; the original training data of the treatment group includes the user's characteristic information, group and user Response under the influence of a given behavior; the original training data of the control group includes user characteristic information, group and user's response under the influence of no given behavior.
[0067] In this step, 80% of the data in the processing group of 1,601,963 transactions and the control group of 603,189 transactions are used to generate training data. Here the final data set will be D={X i ,Y i (1), Y i (0),T i},X i Repres...
Embodiment 2
[0093] Based on the training data generated in embodiment 1, this embodiment further learns a final task learner. The input of the task learner is the user's feature information, and the output is the difference of the user's causal effect and heterogeneous response. Here, two task sub-learners are designed to constitute the final task learner, and the two task sub-learners learn the corresponding training data of the generated control group and treatment group respectively. The two task sub-learner models in this embodiment adopt the same model as the two basic learners in Embodiment 1, namely GRU.
[0094] This step specifically includes the following sub-steps:
[0095] B11 divides the generated training data into two groups: the user characteristic information from the control group and the corresponding causal effect heterogeneous response difference form the control group to generate training data, namely The user characteristic information from the treatment group an...
PUM
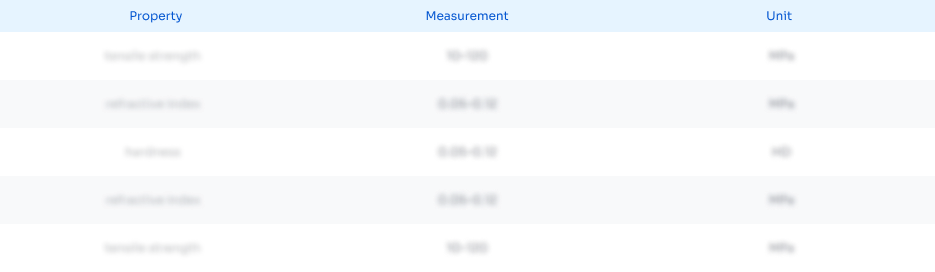
Abstract
Description
Claims
Application Information
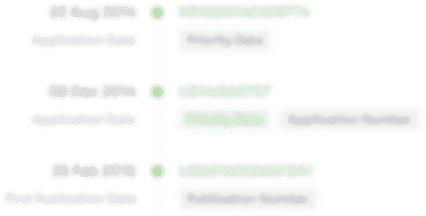
- R&D
- Intellectual Property
- Life Sciences
- Materials
- Tech Scout
- Unparalleled Data Quality
- Higher Quality Content
- 60% Fewer Hallucinations
Browse by: Latest US Patents, China's latest patents, Technical Efficacy Thesaurus, Application Domain, Technology Topic, Popular Technical Reports.
© 2025 PatSnap. All rights reserved.Legal|Privacy policy|Modern Slavery Act Transparency Statement|Sitemap|About US| Contact US: help@patsnap.com