Electrocardiogram anomaly detection method based on VQ-VAE2 and deep neural network method
A technology of VQ-VAE2 and deep neural network, which is applied in diagnostic recording/measurement, medical science, sensors, etc., can solve problems such as sample imbalance, lack of metrics, and poor performance of deep learning models, so as to improve capabilities and improve The effect of accuracy and efficiency
- Summary
- Abstract
- Description
- Claims
- Application Information
AI Technical Summary
Problems solved by technology
Method used
Image
Examples
Embodiment
[0058] like figure 1 shown, including the following steps:
[0059] Step 1: Obtain two training databases, an atrial fibrillation training database and a non-atrial fibrillation training database, and perform data processing on the atrial fibrillation training database;
[0060] Said step 1 includes the following sub-steps:
[0061] 1-1: Obtain two training databases: atrial fibrillation training database and non-atrial fibrillation training database. Trembling ECG data;
[0062] 1-2: Perform denoising processing on each lead ECG signal in the atrial fibrillation training database; the denoising processing on the lead ECG signal in step 1-2 includes the following processing:
[0063] Use median filter to filter out the limit drift in the original ECG signal;
[0064] Use Butterworth digital band-stop filter to filter out the power frequency interference in the original ECG signal;
[0065] A Chebyshev digital low-pass filter was used to filter out myoelectric interference...
PUM
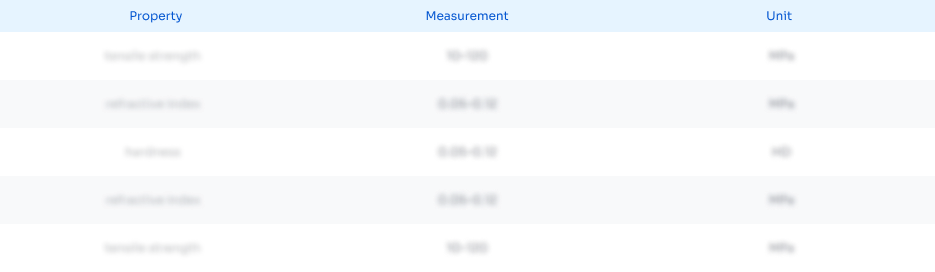
Abstract
Description
Claims
Application Information
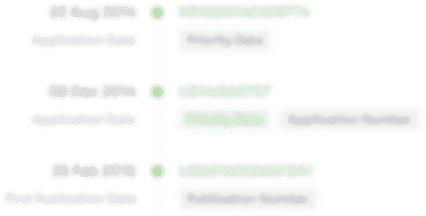
- R&D
- Intellectual Property
- Life Sciences
- Materials
- Tech Scout
- Unparalleled Data Quality
- Higher Quality Content
- 60% Fewer Hallucinations
Browse by: Latest US Patents, China's latest patents, Technical Efficacy Thesaurus, Application Domain, Technology Topic, Popular Technical Reports.
© 2025 PatSnap. All rights reserved.Legal|Privacy policy|Modern Slavery Act Transparency Statement|Sitemap|About US| Contact US: help@patsnap.com