Electroencephalogram-electromyographic signal fusion recognition method based on deep learning
A technology of electromyographic signal and fusion recognition, applied in the field of EEG-EMG signal fusion recognition based on deep learning, can solve the problems of single application field and simple interaction mode, and achieve the effect of improving recognition accuracy and robust fusion
- Summary
- Abstract
- Description
- Claims
- Application Information
AI Technical Summary
Problems solved by technology
Method used
Image
Examples
Embodiment Construction
[0050] The present invention will be described in detail below with reference to the accompanying drawings and specific embodiments.
[0051] The embodiment of the present invention provides a method for fusion recognition of EEG-EMG signal features based on deep learning, such as figure 1 , 2 As shown, the method is specifically implemented through the following steps:
[0052] Step 101: Perform high-dimensional encoding on the preprocessed EEG signals through a recurrent neural network based on a deep self-attention mechanism.
[0053] Specifically, the EEG signal input sequence is processed at regular intervals through a temporal convolutional network based on hole convolution, and the stride is set to be greater than one sample, effectively down-sampling the generated output sequence, and generating a set of effective The filtered sequence after downsampling. Input the sequence of features to the encoder recurrent neural network. The encoder consists of a stack of N id...
PUM
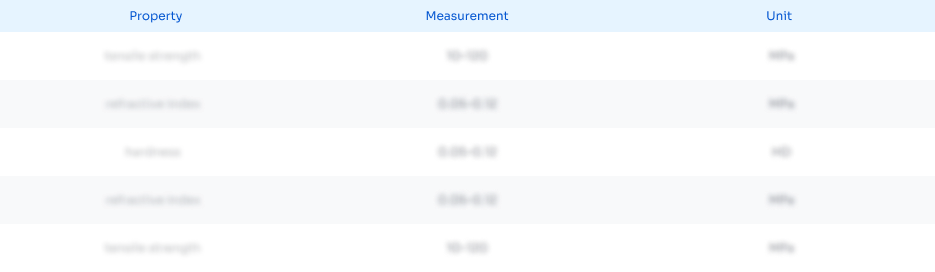
Abstract
Description
Claims
Application Information
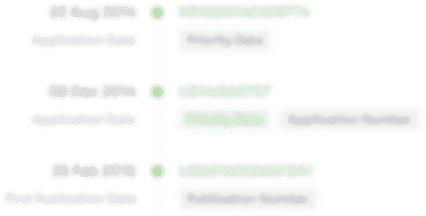
- R&D Engineer
- R&D Manager
- IP Professional
- Industry Leading Data Capabilities
- Powerful AI technology
- Patent DNA Extraction
Browse by: Latest US Patents, China's latest patents, Technical Efficacy Thesaurus, Application Domain, Technology Topic.
© 2024 PatSnap. All rights reserved.Legal|Privacy policy|Modern Slavery Act Transparency Statement|Sitemap