Identification network classification node self-increasing method
A technology for identifying networks and nodes, applied in neural learning methods, character and pattern recognition, biological neural network models, etc., can solve the problem of not being able to add new classification nodes immediately
- Summary
- Abstract
- Description
- Claims
- Application Information
AI Technical Summary
Problems solved by technology
Method used
Image
Examples
Embodiment 1
[0045] Such as figure 1 As shown, a self-incrementing method for identifying network classification nodes comprises the following steps: comprising the following steps:
[0046] S1. Divide the input data stream into several data streams according to the sensor attributes;
[0047] S2. Perform data preprocessing on the divided data stream;
[0048] S3 and T0 are cleared and start timing;
[0049] S4 and T1 are cleared and start timing;
[0050] S5. The identification network corresponding to the data stream input, and judge whether there is a corresponding classification node. If so, clear the corresponding input cumulative value n of no node and proceed to step S10. Otherwise, the value of the cumulative value n of the corresponding input times of no node is increased by 1, and run the step. S6;
[0051] S6. Determine whether the input cumulative value is greater than or equal to the threshold a, if so, proceed to step S5, otherwise proceed to step S7;
[0052] S7. The re...
PUM
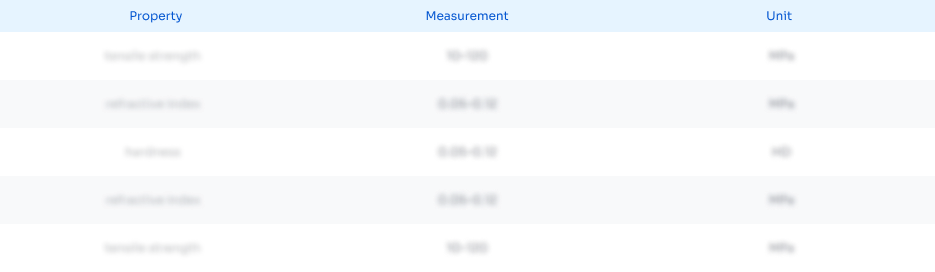
Abstract
Description
Claims
Application Information
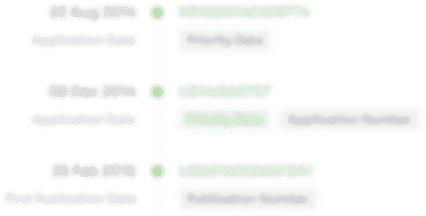
- R&D Engineer
- R&D Manager
- IP Professional
- Industry Leading Data Capabilities
- Powerful AI technology
- Patent DNA Extraction
Browse by: Latest US Patents, China's latest patents, Technical Efficacy Thesaurus, Application Domain, Technology Topic, Popular Technical Reports.
© 2024 PatSnap. All rights reserved.Legal|Privacy policy|Modern Slavery Act Transparency Statement|Sitemap|About US| Contact US: help@patsnap.com