Deep learning-based point-by-point classification fault detection method
A fault detection and deep learning technology, applied in neural learning methods, instruments, biological neural network models, etc., can solve problems such as inability to make full use of timing information, not knowing whether the fault is over, and reasonable disposal of unfavorable faults.
- Summary
- Abstract
- Description
- Claims
- Application Information
AI Technical Summary
Problems solved by technology
Method used
Image
Examples
Embodiment Construction
[0036] In order to make the purpose, technical solution and advantages of the present application clearer, the present application will be further described in detail below in conjunction with the accompanying drawings and embodiments. It should be understood that the specific embodiments described here are only used to explain the present application, and are not intended to limit the present application.
[0037]A deep-learning based Point-wise Classification for Fault Detection (DPCFD) method provided by this application can be applied to complex large-scale industrial systems and is an important means to reduce major accidents.
[0038] In one embodiment, such as figure 1 As shown, a fault detection method based on deep learning point-by-point classification is provided, including the following steps:
[0039] Step 1: Collect the data of each channel of the industrial system to obtain the original data sequence;
[0040] Step 2: Input the original data sequence into the ...
PUM
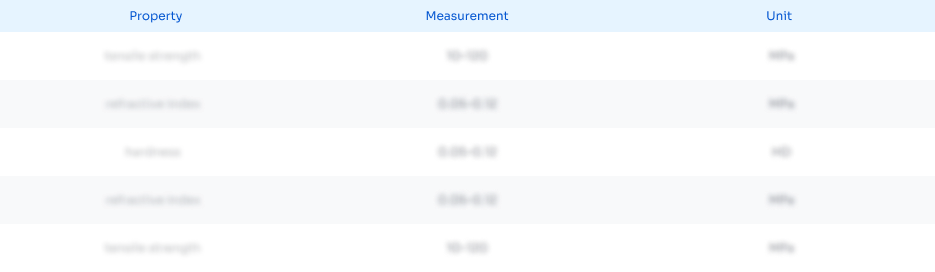
Abstract
Description
Claims
Application Information
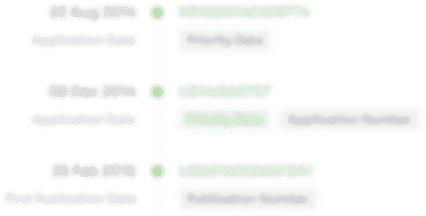
- Generate Ideas
- Intellectual Property
- Life Sciences
- Materials
- Tech Scout
- Unparalleled Data Quality
- Higher Quality Content
- 60% Fewer Hallucinations
Browse by: Latest US Patents, China's latest patents, Technical Efficacy Thesaurus, Application Domain, Technology Topic, Popular Technical Reports.
© 2025 PatSnap. All rights reserved.Legal|Privacy policy|Modern Slavery Act Transparency Statement|Sitemap|About US| Contact US: help@patsnap.com