Dental CBCT three-dimensional tooth segmentation method based on deep learning
A deep learning and dental technology, applied in neural learning methods, dental radiological diagnosis, and instruments for radiological diagnosis, etc., can solve the problem that the processing effect cannot meet the dental diagnosis and other problems
- Summary
- Abstract
- Description
- Claims
- Application Information
AI Technical Summary
Problems solved by technology
Method used
Image
Examples
Embodiment Construction
[0040] The present invention will be further described below in conjunction with drawings and embodiments.
[0041] Such as figure 1 Shown, a deep learning based dental CBCT 3D tooth segmentation method.
[0042]For the tooth segmentation problem of dental CBCT images, the method adopted in the present invention is to perform semantic segmentation on the teeth in each image in the CBCT image sequence, thereby removing noise. To this end, we constructed a deeply supervised encoding-decoding network for denoising oral CT images. The sub-modules of encoding and decoding are connected to each other through a series of nested dense skip paths. The purpose of designing this skip connection is to Reduce the semantic loss of feature maps in the encoding and decoding submodules. The specific steps include: 1. Collection and preprocessing of dental CBCT images; 2. Construction of model training set; 3. Construction of network segmentation model with encoding-decoding structure; 4. Mod...
PUM
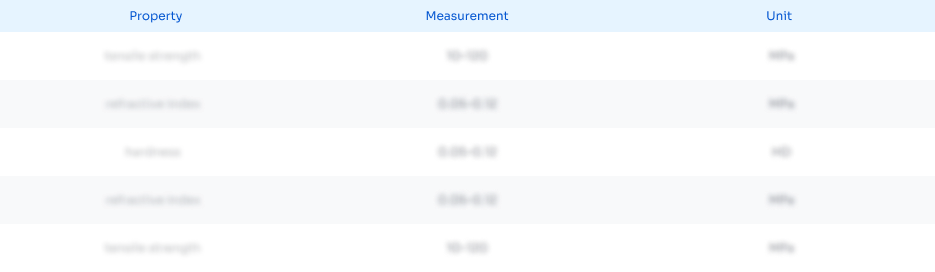
Abstract
Description
Claims
Application Information
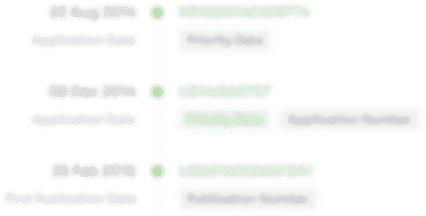
- R&D Engineer
- R&D Manager
- IP Professional
- Industry Leading Data Capabilities
- Powerful AI technology
- Patent DNA Extraction
Browse by: Latest US Patents, China's latest patents, Technical Efficacy Thesaurus, Application Domain, Technology Topic, Popular Technical Reports.
© 2024 PatSnap. All rights reserved.Legal|Privacy policy|Modern Slavery Act Transparency Statement|Sitemap|About US| Contact US: help@patsnap.com