Face feature extraction method, low-resolution face recognition method and device
An extraction method and face feature technology, applied in the field of artificial intelligence, can solve the problems of low accuracy of face image recognition, low resolution of face image, etc., and achieve the effect of efficient follow-up processing and reducing the amount of parameters
- Summary
- Abstract
- Description
- Claims
- Application Information
AI Technical Summary
Problems solved by technology
Method used
Image
Examples
Embodiment 1
[0056] according to figure 1 The structure is built into the feature extracting network, wherein the initial feature extraction module 31 is 3 * 3 convolutional layer, the number of channels of the input and output initial feature extraction module 31 is 3 and 64, respectively, and the length and width of the image is unchanged. . The structure of the GTFB module 32 is like figure 2 As shown in the GTFB module 32, there is a residual connection, and the number of GTFB modules 32 is 6. In the GTFB module 32, the number of channels of input and output 3 * 3, 5 * 5 and the deformable convolutionary feature is equal to 64, 3 * 3 convolution and the deformable convolutionary output feature graph, and pass through 1 * 1 volume and activation function, the output channel is 64, the number of channels of the GTFB module 32 is 192, the number of channels of the volume is 192, the number of channels of the output is 64, such input and output the characteristic map of the GTFB module 32. The...
Embodiment 2
[0063] Based on the network in Example 1, the WSA Care Module 5 is added. For the internal portion of the WSA Care Module 5, the number of feature map channels output by 1 * 1 and the GTFB module 32 is first degraded to 1, and then the various characteristics obtained after the dimension are spliced, and then The number of feature drawings after 1 * 1 is reduced to 1, and after the SIGMOID function is activated, the space is given.
[0064]The comparative experiment is performed using the identical training and test conditions (including experimental methods and steps, hardware, frame, data set, optimizer, learning rate, etc.), and the results showed that the WSA attention module 5 was increased. For the 14 * 14 resolution FERET dataset, the correct rate of identification is 95.3%, and the correct rate of the recognition is 97.1% for the FERET dataset of 28 * 28 resolution. Note The WSA Care Module 5 has a good role in promoting the extraction of face characteristics and face reco...
Embodiment 3
[0066] On the basis of the network in Example 2, the hopping fusion module 6 is increased, and the feature extracts the network structure, such as Figure 4 Indicated. In the present embodiment, the hip fusion module 6 internal structure is Image 6 Indicated. After the first feature, the second feature inputs the jump fusion module 6, both first in the channel direction, obtain a first jump feature map of the number of channels 128. On the other hand, the first feature and the second feature are respectively passed through 3 * 3 convolution and the RELU activation function, respectively generate a second jump feature map and a third jump feature map of the channel number of 128, respectively. Then, the first jump feature, the second jump feature map, and the third hopping feature map are passed through element and fuse, and then pass through the 3 * 3 convolution and the RELU activation function, the output channel number is 128. . The final jump feature map and the feature map out...
PUM
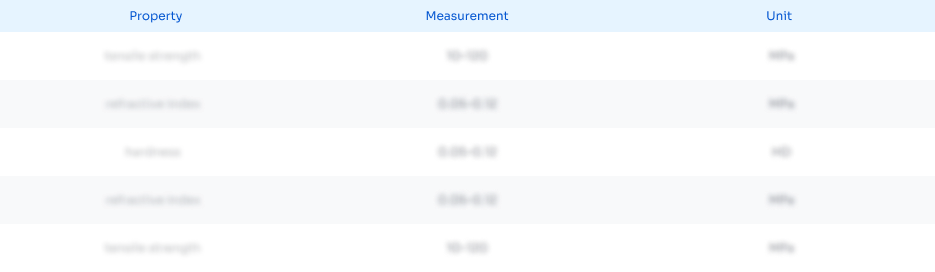
Abstract
Description
Claims
Application Information
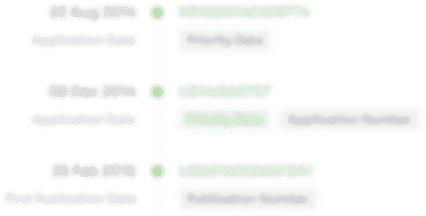
- R&D
- Intellectual Property
- Life Sciences
- Materials
- Tech Scout
- Unparalleled Data Quality
- Higher Quality Content
- 60% Fewer Hallucinations
Browse by: Latest US Patents, China's latest patents, Technical Efficacy Thesaurus, Application Domain, Technology Topic, Popular Technical Reports.
© 2025 PatSnap. All rights reserved.Legal|Privacy policy|Modern Slavery Act Transparency Statement|Sitemap|About US| Contact US: help@patsnap.com