Face feature extraction method, low-resolution face recognition method and device
An extraction method and face feature technology, applied in the field of artificial intelligence, can solve the problems of low resolution of face images and low accuracy of face image recognition, and achieve the effect of efficient follow-up processing and reducing the amount of parameters
- Summary
- Abstract
- Description
- Claims
- Application Information
AI Technical Summary
Problems solved by technology
Method used
Image
Examples
Embodiment 1
[0056] according to figure 1 The shown structure builds a feature extraction network, in which the initial feature extraction module 31 is a 3*3 convolutional layer, the number of channels of the input and output images of the initial feature extraction module 31 are 3 and 64 respectively, and the length and width of the image remain unchanged. . The structure of GTFB module 32 is as follows figure 2 As shown, there are residual connections in the GTFB module 32, and the number of GTFB modules 32 is six. In GTFB module 32, the number of channels of input and output 3*3, 5*5 and deformable convolution feature maps are both 64, 3*3 convolution and deformable convolution output feature maps are added, and after 1* After 1 convolution and activation function, the output channel is a feature map of 64, the number of channels input to the 1*1 convolution layer at the tail of GTFB module 32 is 192, and the number of output channels is 64, so the feature map of GTFB module 32 is in...
Embodiment 2
[0063] On the basis of the network in Embodiment 1, a WSA attention module 5 is added. For the inside of the WSA attention module 5, the first feature map and the number of feature map channels output by the GTFB module 32 are reduced to 1 by using a 1*1 convolutional layer, and then the feature maps obtained after dimensionality reduction are spliced. The 1*1 convolutional layer is used to reduce the number of spliced feature map channels to 1, and after activation by the sigmoid function, the spatial attention map is obtained.
[0064]Using the exact same training and testing conditions (including experimental methods and steps, hardware, framework, data set, optimizer, learning rate, etc.) as in Example 1, a comparative experiment was conducted. The results show that after adding the WSA attention module 5, For the 14*14 resolution FERET dataset, the recognition accuracy is 95.3%, and for the 28*28 resolution FERET dataset, the recognition accuracy is 97.1%. It shows tha...
Embodiment 3
[0066] On the basis of the network in Embodiment 2, a jump connection fusion module 6 is added, and the obtained feature extraction network structure is as follows Figure 4 shown. In this embodiment, the internal structure of the jump connection fusion module 6 is as follows Image 6 shown. After the first feature map and the second feature map are input into the jump connection fusion module 6, they are first spliced in the channel direction to obtain the first jump connection feature map with 128 channels. On the other hand, the first feature map and the second feature map are respectively subjected to 3*3 convolution and ReLU activation function to generate the second hop-connected feature map and the third hop-connected feature map with 128 channels, respectively. Then, the first hop-connected feature map, the second hop-connected feature map, and the third hop-connected feature map are summed and fused through the elements, and after 3*3 convolution and ReLU activati...
PUM
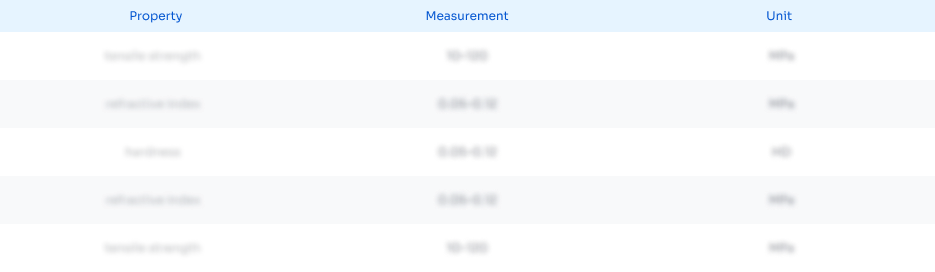
Abstract
Description
Claims
Application Information
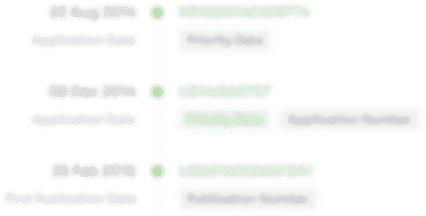
- R&D Engineer
- R&D Manager
- IP Professional
- Industry Leading Data Capabilities
- Powerful AI technology
- Patent DNA Extraction
Browse by: Latest US Patents, China's latest patents, Technical Efficacy Thesaurus, Application Domain, Technology Topic, Popular Technical Reports.
© 2024 PatSnap. All rights reserved.Legal|Privacy policy|Modern Slavery Act Transparency Statement|Sitemap|About US| Contact US: help@patsnap.com