Multidirectional traffic flow prediction method based on interest point space-time residual neural network
A neural network and traffic flow technology, which is applied in the multi-directional traffic flow prediction field based on the spatiotemporal residual neural network of points of interest, can solve problems such as the increase of observation values, and achieve the effect of improving accuracy and strengthening geographical features.
- Summary
- Abstract
- Description
- Claims
- Application Information
AI Technical Summary
Problems solved by technology
Method used
Image
Examples
Embodiment
[0118] The original data used in this example is the real battery car trajectory data set in Zhengzhou. Through preprocessing, each battery car track record retains five fields: vehicle number, longitude, latitude, direction and time. So far, many pieces of data σ= have been formed, and the time unit is minute. Map each σ to the map of each time according to time. Rasterize the map with a size of 400m*400m per square. Count the sum of all vehicles in the same driving state in each grid, and then count the sum of interest points in the same group in each grid, generate traffic flow matrix and interest point signals respectively, and then process the time to be predicted into time signals. Finally, the traffic flow matrix, interest point signal and time signal are input into the model for training according to the above method, and the trained model is used for result prediction. As shown in Figure 1, the multi-directional traffic flow prediction method based on the spatial-t...
PUM
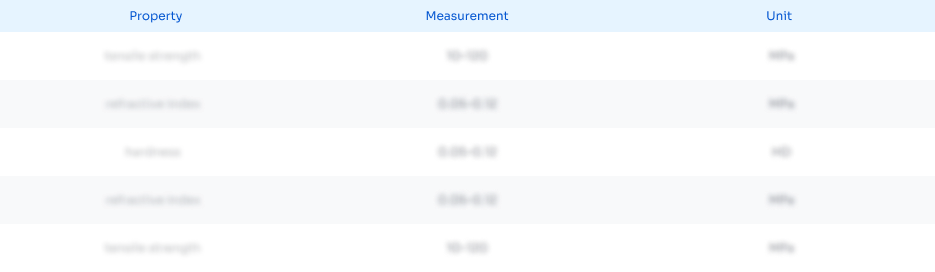
Abstract
Description
Claims
Application Information
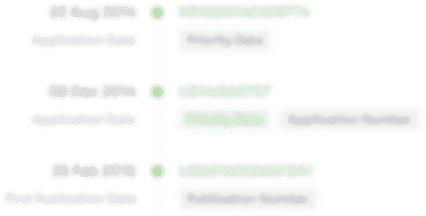
- R&D Engineer
- R&D Manager
- IP Professional
- Industry Leading Data Capabilities
- Powerful AI technology
- Patent DNA Extraction
Browse by: Latest US Patents, China's latest patents, Technical Efficacy Thesaurus, Application Domain, Technology Topic, Popular Technical Reports.
© 2024 PatSnap. All rights reserved.Legal|Privacy policy|Modern Slavery Act Transparency Statement|Sitemap|About US| Contact US: help@patsnap.com