Relational reinforcement learning system and method based on graph structure data
A reinforcement learning, relational technology, applied in the field of deep learning, can solve uninvolved problems and achieve good visual effects
- Summary
- Abstract
- Description
- Claims
- Application Information
AI Technical Summary
Problems solved by technology
Method used
Image
Examples
Embodiment 1
[0050] like figure 1 As shown, a relational reinforcement learning system based on graph-structured data includes:
[0051] Degradation feature extraction module, input the low-resolution image and high-resolution image into the degradation feature extraction module for training, and obtain the image degradation feature encoder;
[0052] The down-sampling module inputs the high-resolution image into the linear down-sampling network, and the generated low-resolution image and the real-world image are then input into the image degradation feature encoder, so that the two can obtain the same degradation features, and at the same time use the color The loss and pixel loss functions ensure that the original infrastructure information of the downsampled image remains unchanged;
[0053] The super-resolution reconstruction module inputs the low-resolution-high-resolution image data generated by the down-sampling module into the super-resolution reconstruction network for training to...
Embodiment 2
[0076] like figure 2 As shown, a learning method for a relational reinforcement learning system based on graph-structured data includes the following steps:
[0077] S1: Input the low-resolution image and high-resolution image into the degradation feature extraction module for training to obtain an image degradation feature encoder;
[0078] S2: Input the high-resolution image into the linear downsampling network, and then input the generated low-resolution image and the real-world image into the encoder trained in step S1, so that the two can obtain the same degradation features, and use The color loss and pixel loss functions ensure that the original infrastructure information of the downsampled image remains unchanged;
[0079] S3: Input the low-resolution-high-resolution image data generated in step S2 into super-resolution reconstruction network training to obtain a real-world image super-resolution model.
[0080] This method encodes real-world images and unmatched hi...
Embodiment 3
[0082] like Figure 1-2 As shown, a learning method for a relational reinforcement learning system based on graph-structured data includes the following steps:
[0083] S1: Input the low-resolution image and high-resolution image into the degradation feature extraction module for training to obtain an image degradation feature encoder;
[0084] S2: Input the high-resolution image into the linear downsampling network, and then input the generated low-resolution image and the real-world image into the encoder trained in step S1, so that the two can obtain the same degradation features, and use The color loss and pixel loss functions ensure that the original infrastructure information of the downsampled image remains unchanged;
[0085] S3: Input the low-resolution-high-resolution image data generated in step S2 into super-resolution reconstruction network training to obtain a real-world image super-resolution model.
[0086] The method is applied to a relational reinforcement ...
PUM
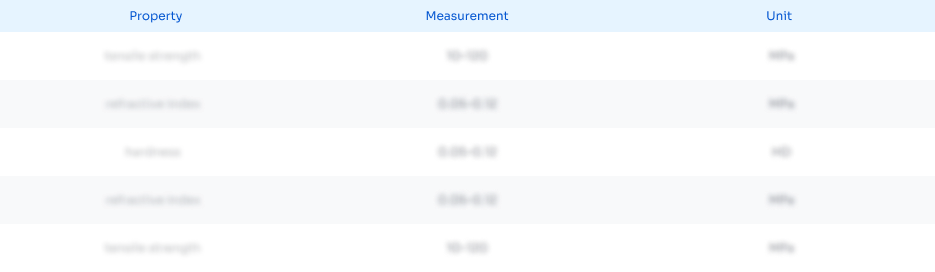
Abstract
Description
Claims
Application Information
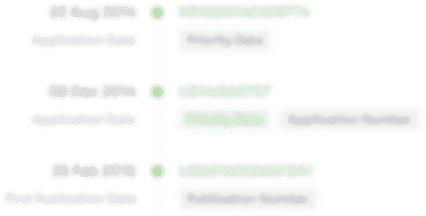
- R&D Engineer
- R&D Manager
- IP Professional
- Industry Leading Data Capabilities
- Powerful AI technology
- Patent DNA Extraction
Browse by: Latest US Patents, China's latest patents, Technical Efficacy Thesaurus, Application Domain, Technology Topic, Popular Technical Reports.
© 2024 PatSnap. All rights reserved.Legal|Privacy policy|Modern Slavery Act Transparency Statement|Sitemap|About US| Contact US: help@patsnap.com