Multi-classification general integration method of adaptive weight
A technology of self-adaptive weight and integration method, applied in the fields of instruments, character and pattern recognition, computer parts, etc., can solve the problems of lack of generality, selection of integration weights, etc., to reduce the amount of calculation, increase the possibility, and increase the stability. sexual effect
- Summary
- Abstract
- Description
- Claims
- Application Information
AI Technical Summary
Problems solved by technology
Method used
Image
Examples
Embodiment approach
[0049] As a possible implementation, this step includes the following sub-steps:
[0050] Step S1031: According to the F of each base model 1 The value is calculated according to the formula (1) and its normalized F 1 value:
[0051]
[0052] Among them, f i F representing the i-th base model 1 value, f i * Denotes the normalized F of the i-th base model 1 value, f min represents the smallest F among all base models 1 value, f max refers to the largest F among all base models 1 value; understandably, but
[0053] Step S1032: According to the normalized F 1 The value calculates the weight of each base model according to the formula (2):
[0054]
[0055] in, w i Represents the weight of the i-th base model, and α represents the weight adjustment coefficient.
[0056] Specifically, in the embodiment of the present invention, on the one hand, an exponential function g(x)=e is introduced x ,Will As a numerator, one can have the weights and the model F ...
PUM
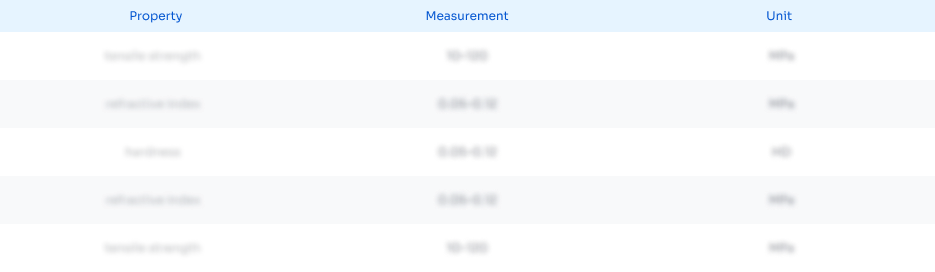
Abstract
Description
Claims
Application Information
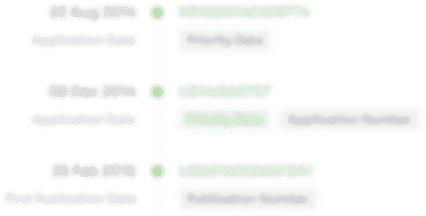
- R&D
- Intellectual Property
- Life Sciences
- Materials
- Tech Scout
- Unparalleled Data Quality
- Higher Quality Content
- 60% Fewer Hallucinations
Browse by: Latest US Patents, China's latest patents, Technical Efficacy Thesaurus, Application Domain, Technology Topic, Popular Technical Reports.
© 2025 PatSnap. All rights reserved.Legal|Privacy policy|Modern Slavery Act Transparency Statement|Sitemap|About US| Contact US: help@patsnap.com