Tea leaf classification method
A classification method, dark green technology, applied in the direction of neural learning methods, instruments, biological neural network models, etc., can solve the problem of strengthening effective features, difficult and complex models to enhance the performance of dark green classification models, and the inability to measure the importance of dark green image matrix channels and the degree of correlation to achieve the effect of reducing the degree of dependence, enhancing the overall performance, and reducing the demand
- Summary
- Abstract
- Description
- Claims
- Application Information
AI Technical Summary
Problems solved by technology
Method used
Image
Examples
Embodiment 1
[0058] refer to Figure 1 to Figure 5 , which is the first embodiment of the present invention, this embodiment provides a green tea classification method combining the ghost attention capsule network and knowledge distillation, the green tea classification method combining the ghost attention capsule network and knowledge distillation includes the following steps:
[0059] S1: dark green image collection;
[0060] S2: Data enhancement and data set establishment;
[0061] S3: Build the ghost attention bottleneck layer and dark green classification model;
[0062] S4: Pre-training and weight acquisition of the ResNet50 model;
[0063] S5: Train the dark green classification model in the way of growing knowledge distillation;
[0064] S6: Performance verification of the tea green classification model.
[0065] Specifically, when performing step S1, a certain amount of green tea green tea is picked from the tea farm, and the tea maker divides it into three categories: single ...
Embodiment 2
[0072] refer to Figure 1 to Figure 9 , is the second embodiment of the present invention, which is based on the previous embodiment.
[0073] Specifically, S1: dark green image acquisition. In this embodiment, an industrial camera with a fixed focal length and aperture is used to shoot dark green on the white base plate, and LED lights are used to supplement light during the process, and image acquisition is ensured. When , the distance between the camera and each sample is constant.
[0074] S2: data enhancement and data set establishment, in this embodiment, by utilizing the argparse library in the Spyder compiler to execute the data enhancement program to complete, the specific process is as follows: set the size of the rectangular frame, and crop the image obtained in step 1, Then the compression operation is performed to limit the size of each picture within 200KB, and finally the tea-green image is expanded by means of geometric transformation, affine transformation, t...
PUM
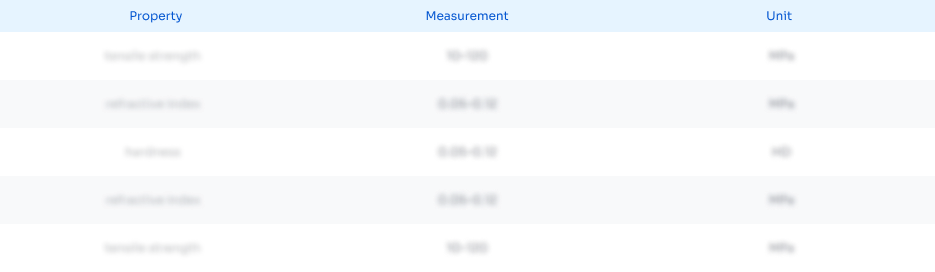
Abstract
Description
Claims
Application Information
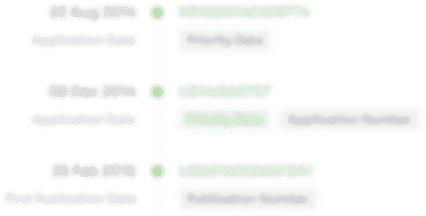
- R&D
- Intellectual Property
- Life Sciences
- Materials
- Tech Scout
- Unparalleled Data Quality
- Higher Quality Content
- 60% Fewer Hallucinations
Browse by: Latest US Patents, China's latest patents, Technical Efficacy Thesaurus, Application Domain, Technology Topic, Popular Technical Reports.
© 2025 PatSnap. All rights reserved.Legal|Privacy policy|Modern Slavery Act Transparency Statement|Sitemap|About US| Contact US: help@patsnap.com