Unsupervised defect detection and positioning method based on multilevel feature reconstruction
A defect detection and positioning method technology, applied in the field of computer vision, can solve the problems of not satisfying the positioning of defect areas of different scales, and the generalization ability is too strong, so as to achieve the effects of cost reduction, strong adaptability and performance improvement
- Summary
- Abstract
- Description
- Claims
- Application Information
AI Technical Summary
Problems solved by technology
Method used
Image
Examples
Embodiment Construction
[0036] The technical solution of the present invention will be specifically described below with reference to the accompanying drawings.
[0037] The non-supervised defect detection and positioning method based on multi-level feature reconstruction, combines the difference between multi-level features and reconstruction features, and realizes detection and positioning of defect samples under conditions without supervising learning. At the same time, the network model is used to calculate the cost of overhead, meet the performance requirements and real-time requirements of defect detection scenes.
[0038] According to a multi-level feature reconstruction, no monitoring defect detection and positioning method, including the following steps:
[0039] Step S1, obtain an abnormal image and abnormal image production data set of the target product;
[0040] Step S2, construct a feature extraction and reconstruction network based on multi-level features;
[0041] Step S3, input the train...
PUM
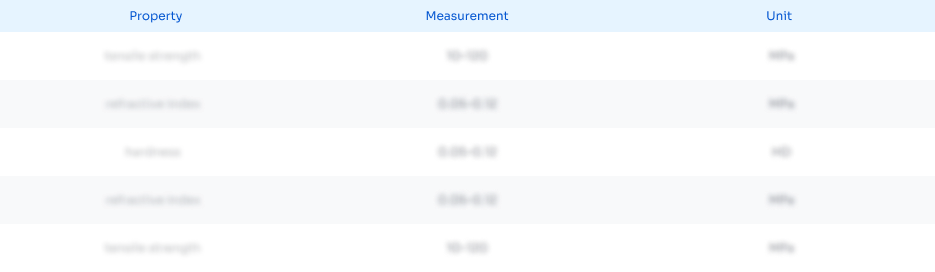
Abstract
Description
Claims
Application Information
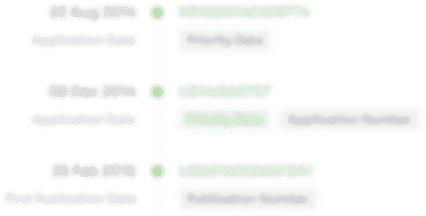
- R&D Engineer
- R&D Manager
- IP Professional
- Industry Leading Data Capabilities
- Powerful AI technology
- Patent DNA Extraction
Browse by: Latest US Patents, China's latest patents, Technical Efficacy Thesaurus, Application Domain, Technology Topic.
© 2024 PatSnap. All rights reserved.Legal|Privacy policy|Modern Slavery Act Transparency Statement|Sitemap