Semi-supervised medical image segmentation method and device based on dual-model interactive learning
A medical image, semi-supervised technology, applied in image analysis, image data processing, biological neural network model, etc., can solve problems such as improving the quality of pseudo-labels, and achieve the effect of simple implementation method, significant segmentation effect, and flexible means.
- Summary
- Abstract
- Description
- Claims
- Application Information
AI Technical Summary
Problems solved by technology
Method used
Image
Examples
Embodiment Construction
[0038] In order to make the object, technical solution and advantages of the present invention clearer, the present invention will be further described in detail below with reference to the accompanying drawings and embodiments. However, it should be understood that the specific embodiments described here are only used to explain the present invention, and are not intended to limit the scope of the present invention. Also, in the following description, descriptions of well-known structures and techniques are omitted to avoid unnecessarily obscuring the concept of the present invention.
[0039] Embodiments of the present invention provide a semi-supervised medical image segmentation method based on dual-model interactive learning, comprising the following steps:
[0040] 1. Experiment setup and preparation:
[0041] The invention mainly solves the problem of improving the quality of pseudo labels and the performance of model segmentation in the semi-supervised medical image s...
PUM
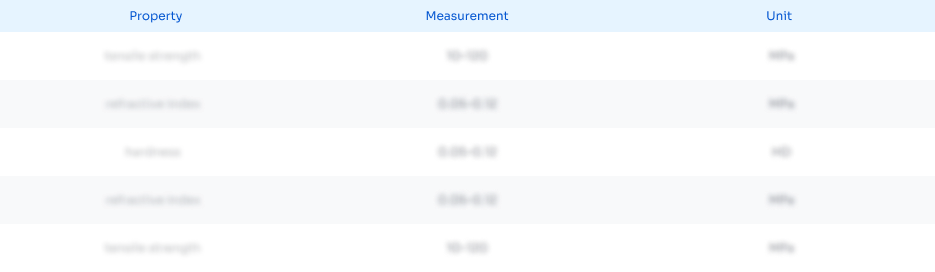
Abstract
Description
Claims
Application Information
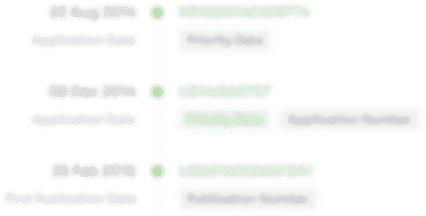
- R&D
- Intellectual Property
- Life Sciences
- Materials
- Tech Scout
- Unparalleled Data Quality
- Higher Quality Content
- 60% Fewer Hallucinations
Browse by: Latest US Patents, China's latest patents, Technical Efficacy Thesaurus, Application Domain, Technology Topic, Popular Technical Reports.
© 2025 PatSnap. All rights reserved.Legal|Privacy policy|Modern Slavery Act Transparency Statement|Sitemap|About US| Contact US: help@patsnap.com