Non-intrusive load identification method based on metric learning
A non-invasive, load identification technology, applied in neural learning methods, character and pattern recognition, instruments, etc., can solve problems such as poor model generalization performance, increased maintenance complexity and cost, and inability to identify new loads, etc., to achieve strong Generalization performance, reduced migration cost, and high practical value
- Summary
- Abstract
- Description
- Claims
- Application Information
AI Technical Summary
Problems solved by technology
Method used
Image
Examples
Embodiment 1
[0083] In order to illustrate the general recognition ability of the present invention, use 80% WHITED data set samples as training set to train feature extraction network, test in the remaining samples, and common V-I method: Literature [1] (De Baets L, Dhaene T, Deschrijver D ,et al.VI-Based Appliance Classification Using Aggregated PowerConsumption Data[C] / / 2018 IEEE International Conference on Smart Computing(SMARTCOMP).Taormina:IEEE,2018:179–186.), Literature [2](Wang Ying, Yang Wei, Xiao Xianyong, Zhang Shu. Non-intrusive Residential Load Monitoring Method Based on Refined Identification of U-I Trajectory Curve [J]. Power Grid Technology, 2021, 45(10): 4104-4113.) and literature [3] (De Baets L ,Ruyssinck J,Develder C,et al.ApplianceClassification Using VI Trajectories and Convolutional Neural Networks[J].Energy and Buildings,2018,158:32–36.) For comparison, use F 1 The scores are used as performance indicators, and the results are shown in Table 1.
[0084] Table 1 Com...
Embodiment 2
[0088] In order to verify the generality and small-sample learning ability of the method of the present invention, only the samples of house6 in the PLAID dataset are used as the training set, and some COOLL datasets are selected for testing.
[0089] The electrical appliances selected in the COOLL data set include air conditioners, modems, chargers, travel chargers, drilling machines, fans 1, fan 2, soldering irons, and vacuum cleaners. Collect 20 sets of information for each electrical appliance as a test sample and get the recognition result. The confusion matrix is attached Figure 6 shown.
[0090] Due to signal noise or current fluctuations during steady-state operation of electrical appliances, some electrical appliances are identified as multiple numbers, as shown in the confusion matrix, electrical appliance 1 and electrical appliance 5 respectively recognize two numbers, that is, the waveform difference within the class exceeds The threshold set by the model, cert...
PUM
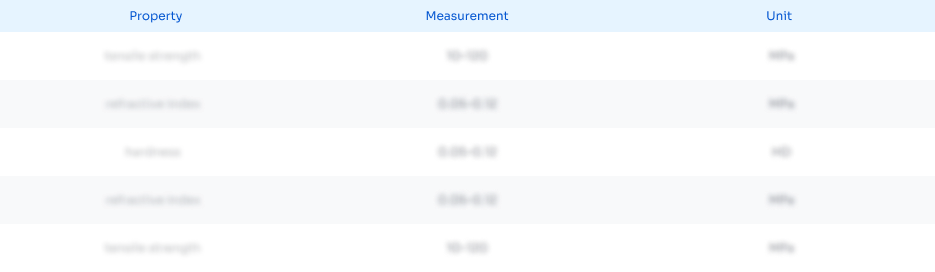
Abstract
Description
Claims
Application Information
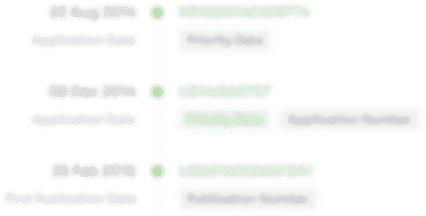
- R&D Engineer
- R&D Manager
- IP Professional
- Industry Leading Data Capabilities
- Powerful AI technology
- Patent DNA Extraction
Browse by: Latest US Patents, China's latest patents, Technical Efficacy Thesaurus, Application Domain, Technology Topic, Popular Technical Reports.
© 2024 PatSnap. All rights reserved.Legal|Privacy policy|Modern Slavery Act Transparency Statement|Sitemap|About US| Contact US: help@patsnap.com