Underwater target classification method considering robustness of deep learning model
An underwater target and deep learning technology, applied in machine learning, computing models, character and pattern recognition, etc., can solve the problem of low classification accuracy of underwater targets, and achieve improved classification accuracy, improved robustness, and accurate classification Effect
- Summary
- Abstract
- Description
- Claims
- Application Information
AI Technical Summary
Problems solved by technology
Method used
Image
Examples
specific Embodiment approach 1
[0056] Specific implementation mode one: combine figure 1 Illustrate this embodiment, a kind of method of underwater target classification that considers deep learning model robustness described in this embodiment, it comprises the following steps:
[0057] S1. Establish an original model, use the collected underwater target data training set to train the original model until convergence, and obtain the trained original model;
[0058] Use the trained original model to predict the collected underwater target data training set, and obtain the set of all correctly classified samples and the set of all misclassified samples;
[0059] Establish the original model, use the collected underwater target data training set to train the original model until it converges, and get the trained original model. The original model can be any deep learning model. Use the trained original model to analyze the collected water The target data training set is used for prediction, and a set of all ...
Embodiment 1
[0112] First establish the original model, use the collected underwater target data training set to train the original model until it converges, and get the trained original model, use the trained original model to predict the underwater target data training set, and get all the correct classifications The set of samples and the set S of all misclassified samples false , then the resulting S false Input the original model again, let the output of the original model be the characteristics of the corresponding misclassified samples, use the K-means clustering method to cluster the characteristics of the misclassified samples, and obtain the corresponding k cluster sets expressed as {KM 1 , KM 2 ,...,KM k}, and there is no overlap between any two clustering sets, the formula can be obtained as follows:
[0113]
[0114] Among them, Card() indicates the number of elements in the set, and Card(S false ) represents the number of all misclassified samples, Card(KM w ) represe...
PUM
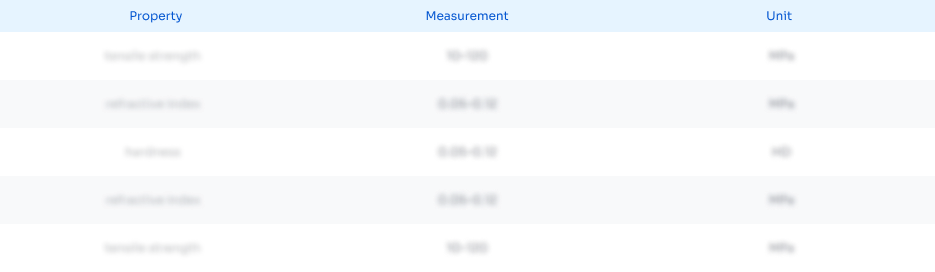
Abstract
Description
Claims
Application Information
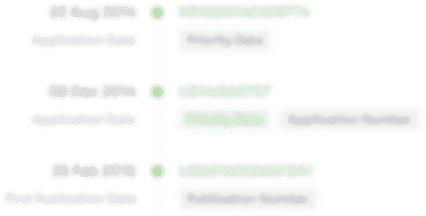
- R&D Engineer
- R&D Manager
- IP Professional
- Industry Leading Data Capabilities
- Powerful AI technology
- Patent DNA Extraction
Browse by: Latest US Patents, China's latest patents, Technical Efficacy Thesaurus, Application Domain, Technology Topic, Popular Technical Reports.
© 2024 PatSnap. All rights reserved.Legal|Privacy policy|Modern Slavery Act Transparency Statement|Sitemap|About US| Contact US: help@patsnap.com