Distributed pattern recognition training method and system
a pattern recognition and distribution pattern technology, applied in the field of distribution pattern recognition training system and method, can solve the problems of large amount of data required for training these speech recognition systems, large amount of data required for speech recognition applications, and enormous quantity of data
- Summary
- Abstract
- Description
- Claims
- Application Information
AI Technical Summary
Problems solved by technology
Method used
Image
Examples
first embodiment
[0050] Secondly, the first embodiment is capable of using a continuing, on-going data collection process. In many applications, this on-going data collection takes place at many, physically separated sites. In such a case, it is more efficient if much of the processing can be done locally at the data collection sites.
[0051] In large scale implementations, each peripheral site and especially the central processing node may themselves be large networked data centers with many processors each. The functional characteristic that distinguishes the peripheral data sites from the central node is that, as part of the first embodiment, peripheral sites do not need to communicate directly with each other, whereby they only need to communicate with the central node. Within any one site or the central node, all the processors within a multiprocessor implementation may communicate with each other without restriction.
[0052] The first embodiment permits a large collection of data observations at ...
second embodiment
[0108] In block 330, in the second embodiment, the functionals φj(.) have already been communicated, so a candidate solution can be specified just by communicating its set of weights {wj}. The central node communicates the weights to the peripheral sites.
[0109] In block 340, a plurality of data items are obtained at each peripheral site. In the second embodiment, data collection is an on-going process, so that in a given iteration there may be new data items at some peripheral sites that were not known during previous iterations.
[0110] In block 350, which is an instance of block 150 from FIG. 1, statistics are computed at the peripheral sites, to be communicated back to the central node. In particular, for each peripheral node P, block 350 computes for the second embodiment shown in FIG. 3 the quantity ∑i∈IP g′(wjφj(x->) yi-di)∂ξi∂wj.(0.7)
[0111] Essentially, expression (0.7) tells the central node the net change in the objective function for a change in the weight wj, s...
third embodiment
[0119] In the third embodiment, the optimization problem takes the form Minimize:E=∑j wj+C∑i ξiSubjectTo:∀i∑j wjai,j+ξi≥di;ξi≥0; ai,j=φj(x->i) yi(0.9)
[0120] Thus, the optimization problem is a (primal) linear programming problem whose dual is given by Maximize:D=∑i diλiSubjectTo: ∀j∑i λiai,j≤ 1; ∀i0≤ λi≤ C;ai,j= φj(x->i) y i(0.10)
[0121] Block 110 of FIG. 4 is the same as block 110 of FIG. 1. It provides for data communication between a central node and a plurality of peripheral sites in order to compute solutions to a series of problems of the form (0.9) and (0.10).
[0122] In block 415, at least one kernel function is communicated from the central node to the peripheral sites. These kernel functions are communicated to the peripheral sites in this embodiment so that each particular peripheral site will be able to form new functionals from the data items obtained at that particular peripheral site.
[0123] In block 420, an initial set of da...
PUM
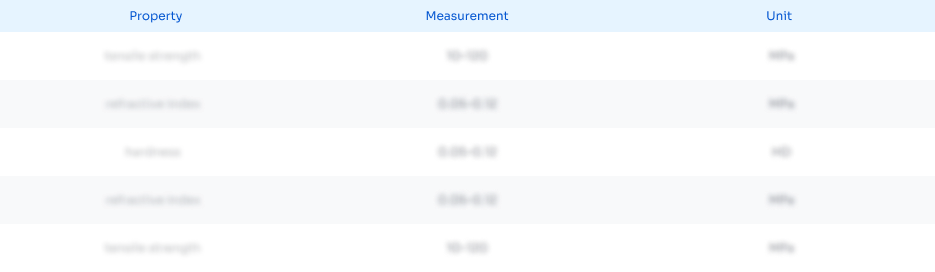
Abstract
Description
Claims
Application Information
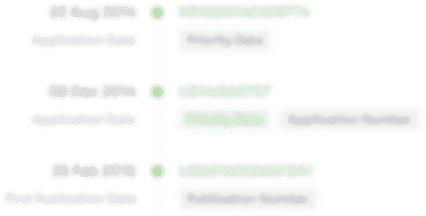
- R&D
- Intellectual Property
- Life Sciences
- Materials
- Tech Scout
- Unparalleled Data Quality
- Higher Quality Content
- 60% Fewer Hallucinations
Browse by: Latest US Patents, China's latest patents, Technical Efficacy Thesaurus, Application Domain, Technology Topic, Popular Technical Reports.
© 2025 PatSnap. All rights reserved.Legal|Privacy policy|Modern Slavery Act Transparency Statement|Sitemap|About US| Contact US: help@patsnap.com