Hydrological time series prediction method based on multiple-factor wavelet neural network model
A hydrological time series, wavelet neural network technology, applied in biological neural network models, special data processing applications, instruments, etc., to achieve high prediction accuracy, good scalability and practical value.
- Summary
- Abstract
- Description
- Claims
- Application Information
AI Technical Summary
Problems solved by technology
Method used
Image
Examples
Embodiment Construction
[0029] The technical scheme of the present invention is described in detail below in conjunction with accompanying drawing:
[0030] The multi-factor wavelet neural network forecasting model of the present invention takes multi-time series information as input, not only includes the current wavelet coefficients of the forecast target time series, but also includes the current wavelet coefficients of other time series related to this time series, and its structure is as follows figure 1 As shown, the site to be predicted is A, and the sites related to A are B to I. This multi-factor wavelet neural network prediction model can also be called the river channel wavelet network model, abbreviated as RWNN.
[0031] The hydrological time series prediction method based on the multi-factor wavelet neural network model of the present invention is carried out according to the following steps:
[0032] Step 10, determining the relevant input time series;
[0033] In information theory, ...
PUM
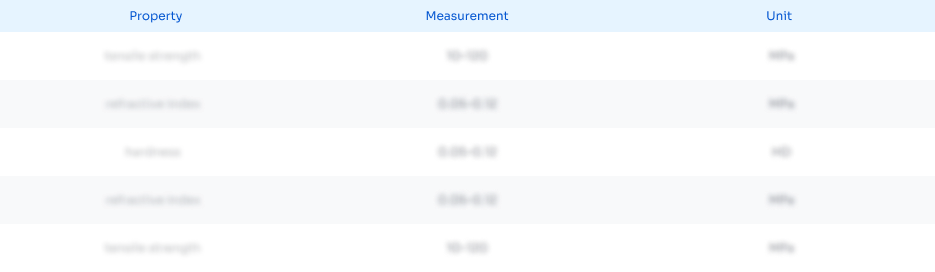
Abstract
Description
Claims
Application Information
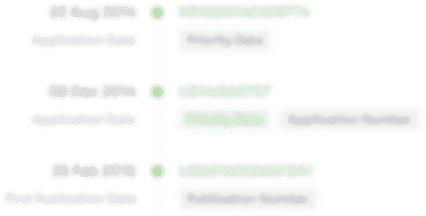
- R&D Engineer
- R&D Manager
- IP Professional
- Industry Leading Data Capabilities
- Powerful AI technology
- Patent DNA Extraction
Browse by: Latest US Patents, China's latest patents, Technical Efficacy Thesaurus, Application Domain, Technology Topic.
© 2024 PatSnap. All rights reserved.Legal|Privacy policy|Modern Slavery Act Transparency Statement|Sitemap