Local and global consistence classifying method based on sparse decomposition of figure 10
A technology of sparse decomposition and classification method, applied in the field of semi-supervised classification based on graph, which can solve the problems of large amount of calculation and inapplicability to obtain the most similar nodes of the graph, and achieve the effect of improving the generalization ability and classification accuracy.
- Summary
- Abstract
- Description
- Claims
- Application Information
AI Technical Summary
Problems solved by technology
Method used
Image
Examples
Embodiment Construction
[0036] Now in conjunction with embodiment, accompanying drawing, the present invention will be further described:
[0037] Step 1: Sparse decomposition l 0 graph construction
[0038] Step a: Use the KMEANS clustering algorithm to divide the entire data X to be classified into k subsets, where k is the number of categories;
[0039] Step b: Define an n×n adjacency matrix W, whose elements are initialized to 0, and n is the total number of data to be classified;
[0040] Step c: Take the i-th data x to be classified i For the signal to be decomposed, the sparse decomposition dictionary D i by x i The cluster subsets to which it belongs, x i not included in D i Among them, 1≤i≤n, n is the total number of data to be classified;
[0041] Step d: The preset maximum number of iterations is T=5, and the iteration termination error is ε stop =0.001, the current iteration number t is initialized to 0, and the decomposition signal x i , based on the dictionary D i use
[0042...
PUM
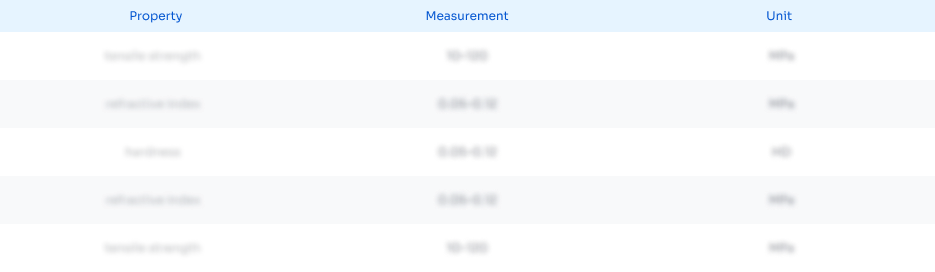
Abstract
Description
Claims
Application Information
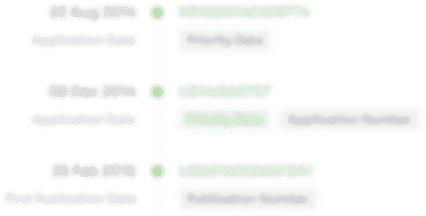
- R&D
- Intellectual Property
- Life Sciences
- Materials
- Tech Scout
- Unparalleled Data Quality
- Higher Quality Content
- 60% Fewer Hallucinations
Browse by: Latest US Patents, China's latest patents, Technical Efficacy Thesaurus, Application Domain, Technology Topic, Popular Technical Reports.
© 2025 PatSnap. All rights reserved.Legal|Privacy policy|Modern Slavery Act Transparency Statement|Sitemap|About US| Contact US: help@patsnap.com