Mode recognition method of mixed linear SVM (support vector machine) classifier with hierarchical structure
A technology of hierarchical structure and pattern recognition, applied in the direction of character and pattern recognition, instruments, computer parts, etc., can solve the problem that SVM cannot handle the classification problem of large amount of data, the classification time complexity is high, and it cannot process classified data, etc. Achieve the effect of overcoming the difficulty of selecting the kernel function and its parameters, improving the generalization ability, and reducing the time complexity of classification
- Summary
- Abstract
- Description
- Claims
- Application Information
AI Technical Summary
Problems solved by technology
Method used
Image
Examples
Embodiment Construction
[0031] The present invention is specifically described below through the examples, only for further illustrating the present invention, can not be interpreted as the limitation of protection scope of the present invention, those skilled in the art can make some non-essential improvements and improvements to the present invention according to the content of the above-mentioned invention Adjustment.
[0032] figure 1 It is a training flowchart of the H-MLSVMs classifier of the present invention, which is a pattern recognition method of a mixed linear SVM classifier with hierarchical structure. figure 2 It is shown as a two-dimensional example of the classifier training process of the present invention. image 3 Flowchart of the classification process for new samples for the H-MLSVMs classifier. The specific operating hardware and programming language of the method of the present invention are not limited, and can be written in any language, so other working modes will not be ...
PUM
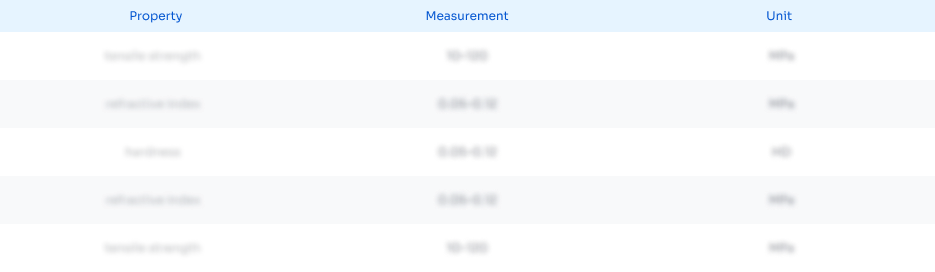
Abstract
Description
Claims
Application Information
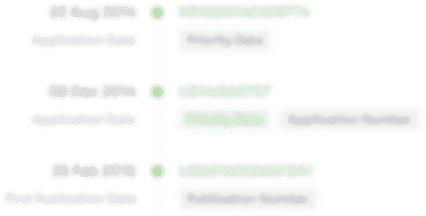
- R&D Engineer
- R&D Manager
- IP Professional
- Industry Leading Data Capabilities
- Powerful AI technology
- Patent DNA Extraction
Browse by: Latest US Patents, China's latest patents, Technical Efficacy Thesaurus, Application Domain, Technology Topic, Popular Technical Reports.
© 2024 PatSnap. All rights reserved.Legal|Privacy policy|Modern Slavery Act Transparency Statement|Sitemap|About US| Contact US: help@patsnap.com