Image feature extraction method based on Laplace operator
A Laplacian operator and image feature extraction technology, applied in the field of image feature extraction based on Laplacian operator, can solve the problem of high cost of data without class labels and class labels
- Summary
- Abstract
- Description
- Claims
- Application Information
AI Technical Summary
Problems solved by technology
Method used
Image
Examples
Embodiment Construction
[0034] With reference to accompanying drawing, further illustrate the present invention:
[0035] A kind of image feature extraction method based on Laplacian, this method comprises the following steps:
[0036] 1) Obtain original image feature data
[0037] Use the Internet to collect images, obtain an image library, and use a high-dimensional vector x for each image according to the visual characteristics of the image i =(f 1,i ,..., f n,i ) T said, f j,i Indicates the value of the j-th feature corresponding to the i-th image.
[0038] 2) Get the Laplacian matrix
[0039] All image feature vectors obtained in all steps 1) are expressed as a matrix X=(x 1 ,...,x m ), where each column vector x i All correspond to the vector obtained from step 1) of the i-th image, and each row vector corresponds to a certain feature. The goal of the method is to extract suitable features, that is, to extract suitable row vectors. use Represents the matrix represented by the last ...
PUM
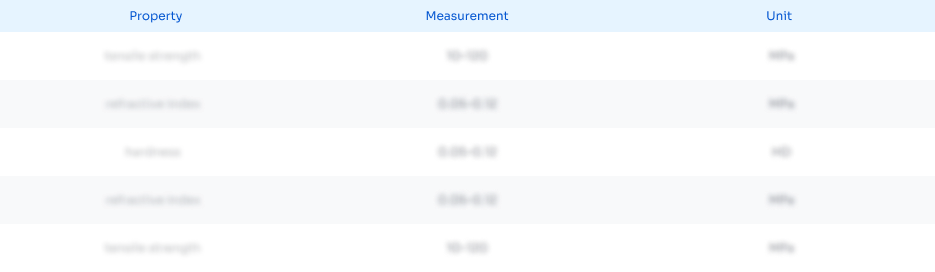
Abstract
Description
Claims
Application Information
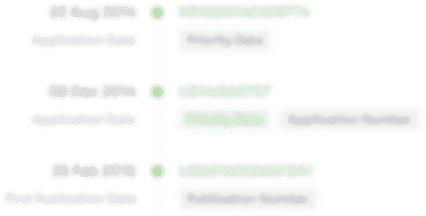
- R&D
- Intellectual Property
- Life Sciences
- Materials
- Tech Scout
- Unparalleled Data Quality
- Higher Quality Content
- 60% Fewer Hallucinations
Browse by: Latest US Patents, China's latest patents, Technical Efficacy Thesaurus, Application Domain, Technology Topic, Popular Technical Reports.
© 2025 PatSnap. All rights reserved.Legal|Privacy policy|Modern Slavery Act Transparency Statement|Sitemap|About US| Contact US: help@patsnap.com