Risk Factors And Prediction Of Myocardial Infarction
A myocardial infarction, risk technology, applied in measurement devices, biological tests, instruments, etc., can solve problems such as imperfection
- Summary
- Abstract
- Description
- Claims
- Application Information
AI Technical Summary
Problems solved by technology
Method used
Image
Examples
Embodiment 1
[0058] Example 1: Identification of putative biomarkers
[0059] method
[0060] research group
[0061] The aim of this study was to improve detection of individuals at highest risk by focusing on individuals who developed MI within 4 years of risk assessment. Risk factors and predictors for this type of recent MI have not been reported previously, mainly because most studies were too small to accrue a sufficient number of recent MI events. Clearly, the risk factors for short-term events like MI, dominated by thrombosis superimposed on inflamed and ruptured atherosclerotic plaques, are different from the risks of longer-term events dominated by the slow progression of atherosclerosis factor. To this end, a large community-based prospective nested case-control study was used, a combination of the Copenhagen General Population Study and the Copenhagen City Heart Study utilizing 45,735 men and women.
[0062] Participants were from the Copenhagen City Heart Study 2001-2003...
Embodiment 2
[0130] The study population, established risk factors, and inferred biomarkers are as described in Example 1. Variables representing established risk factors were treated as in Example 1, and variables representing inferred biomarkers were treated as continuous variables.
[0131] Associations between baseline variable levels and MI incidence were examined with logistic regression models. The model included the 13 biomarkers identified in Example 1 and listed in Table 6.
[0132] An exemplary equation for determining a risk score indicative of a person's risk of developing MI is calculated by multiplying the measured level of each biomarker by a coefficient reflecting its relative contribution to risk, and linearizing each product and , to produce a risk score. The measured levels of each biomarker were natural logarithmically transformed (ie, base e, where e is about 2.71828183) before multiplication by the coefficient. As will be appreciated by those skilled in the art, a...
Embodiment 3
[0144] The study population, established risk factors, and inferred biomarkers are as described in Example 1. Variables representing established risk factors were treated as described in Example 1, and variables representing inferred biomarkers were treated as continuous variables.
[0145] Associations between baseline variable levels and MI incidence were examined with logistic regression models. This example initially considered the 13 biomarkers identified in Example 1 (same starting set as used in Example 2 above).
[0146] To select a subset of informative biomarkers from this initial 13 biomarkers, a backward stepwise variable selection technique was used. At each stepwise selection step, a biomarker was retained if the p-value associated with its coefficient was p<0.05. In this way, 7 biomarkers were retained for the final model. Table 8 lists their coefficients:
[0147] Table 8: Biomarker Coefficients of Example 3
[0148] Biomarkers
Coefficient
...
PUM
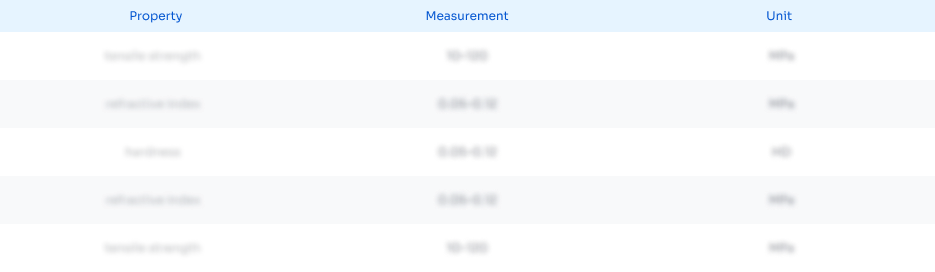
Abstract
Description
Claims
Application Information
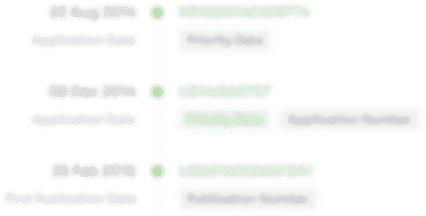
- R&D
- Intellectual Property
- Life Sciences
- Materials
- Tech Scout
- Unparalleled Data Quality
- Higher Quality Content
- 60% Fewer Hallucinations
Browse by: Latest US Patents, China's latest patents, Technical Efficacy Thesaurus, Application Domain, Technology Topic, Popular Technical Reports.
© 2025 PatSnap. All rights reserved.Legal|Privacy policy|Modern Slavery Act Transparency Statement|Sitemap|About US| Contact US: help@patsnap.com