Optimal training method of collaborative filtering recommendation model
A collaborative filtering recommendation and training method technology, applied in the direction of computing models, knowledge-based computer systems, instruments, etc., can solve the problems of interdependence between latent features of users and latent features of items, inability to parallelize, limit model promotion, etc. , to improve scalability, improve build speed, and eliminate interdependencies
- Summary
- Abstract
- Description
- Claims
- Application Information
AI Technical Summary
Problems solved by technology
Method used
Image
Examples
Embodiment 1
[0026] Embodiment one: if figure 1 As shown, a method for optimizing training of a collaborative filtering recommendation model is characterized in that it proceeds in the following steps:
[0027] Step 1, a single-column user latent feature matrix;
[0028] For the matrix factorization recommendation model that needs to be constructed, its user latent feature matrix P is single-columned;
[0029] Step 2: Determine whether the latent feature matrix is converged; when the user latent feature matrix is converged, output the user latent feature matrix after training; when the user latent feature matrix is not converged, perform step 3.
[0030] Step 3. Construct a user hidden feature vector training process based on single-column stochastic gradient descent;
[0031] According to the single-column user latent feature matrix, decompose the latent feature matrix training process of the matrix factorization recommendation model to obtain the training sub-process of the user ...
Embodiment 2
[0035] Embodiment two: if figure 2 As shown, an optimal training method for a collaborative filtering recommendation model is carried out in the following steps:
[0036] Step 1, a single-column item latent feature matrix;
[0037] For the matrix factorization recommendation model that needs to be constructed, its item latent feature matrix Q is single-columned;
[0038] Step 2. Determine whether the latent feature matrix is converged; when the latent feature matrix of the item is converged, output the latent feature matrix of the item after training; when the latent feature matrix of the item is not converged, perform step 3.
[0039] Step 3. Construct the training process of item latent feature vectors based on single-column stochastic gradient descent;
[0040] According to the single-column item latent feature matrix, decompose the latent feature matrix training process of the matrix factorization recommendation model to obtain the training sub-process of the item laten...
Embodiment 3
[0044] Embodiment three: as image 3 As shown, an optimal training method for a collaborative filtering recommendation model is carried out in the following steps:
[0045] Step 1, single-column user latent feature matrix and item latent feature matrix;
[0046] For the matrix factorization recommendation model that needs to be constructed, its user latent feature matrix P and item latent feature matrix Q are listed separately;
[0047] Step 2. Determine whether the user latent feature matrix and the item latent feature matrix are converged; when the user latent feature matrix and the item latent feature matrix converge, output the user latent feature matrix and the item latent feature matrix that have been trained; when the user latent feature matrix or the item When the latent feature matrix is not converged, go to step 3.
[0048] Step 3. Construct a user hidden feature vector and item hidden feature vector training process based on single-column stochastic gradient des...
PUM
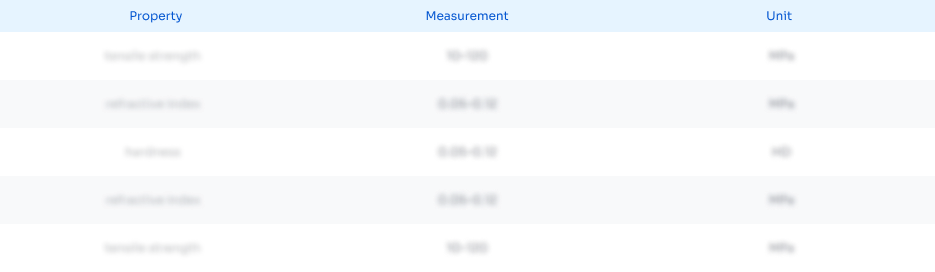
Abstract
Description
Claims
Application Information
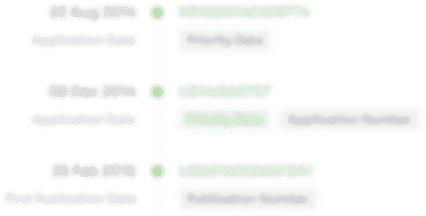
- R&D Engineer
- R&D Manager
- IP Professional
- Industry Leading Data Capabilities
- Powerful AI technology
- Patent DNA Extraction
Browse by: Latest US Patents, China's latest patents, Technical Efficacy Thesaurus, Application Domain, Technology Topic, Popular Technical Reports.
© 2024 PatSnap. All rights reserved.Legal|Privacy policy|Modern Slavery Act Transparency Statement|Sitemap|About US| Contact US: help@patsnap.com