Training sample grouping construction method used for support vector regression (SVR) short-term load forecasting
A technology of short-term load forecasting and training samples, applied in special data processing applications, instruments, electrical digital data processing, etc., can solve problems such as poor reliability, less research, and unsatisfactory training samples, so as to improve load forecasting accuracy and avoid time-consuming The effect of high complexity
- Summary
- Abstract
- Description
- Claims
- Application Information
AI Technical Summary
Problems solved by technology
Method used
Image
Examples
Embodiment Construction
[0018] The present invention will be further described below in conjunction with the accompanying drawings and specific embodiments.
[0019] figure 1 It is a schematic diagram of the present invention. refer to figure 1 As shown, the present invention first analyzes the Dunn's correlation between each time interval and all other time intervals; then, according to the calculated correlation, the prediction problem is grouped according to the time intervals to solve the problem that the proportion of certain types of data in the entire data set is too small problem with a huge sample size; then, construct a reference load matrix including simulated forecast load and reference load for each group, and use the reference load matrix to construct a load change rate matrix; finally, use the load change rate matrix to calculate the Fitting variance, according to the fitting variance, select reference loads to construct training samples for each group of questions.
[0020] It is a...
PUM
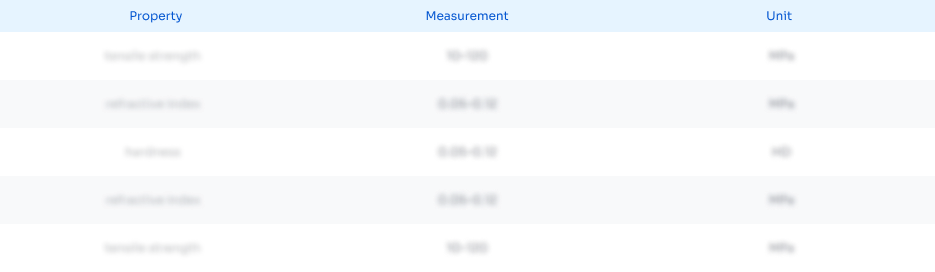
Abstract
Description
Claims
Application Information
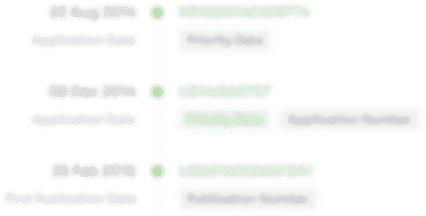
- R&D
- Intellectual Property
- Life Sciences
- Materials
- Tech Scout
- Unparalleled Data Quality
- Higher Quality Content
- 60% Fewer Hallucinations
Browse by: Latest US Patents, China's latest patents, Technical Efficacy Thesaurus, Application Domain, Technology Topic, Popular Technical Reports.
© 2025 PatSnap. All rights reserved.Legal|Privacy policy|Modern Slavery Act Transparency Statement|Sitemap|About US| Contact US: help@patsnap.com