A speech emotion recognition method based on semi-supervised feature selection
A speech emotion recognition and feature selection technology, applied in speech analysis, instruments, etc., can solve problems such as difficult occurrence of labeled samples, feature differences, and large speaker differences
- Summary
- Abstract
- Description
- Claims
- Application Information
AI Technical Summary
Problems solved by technology
Method used
Image
Examples
Embodiment 1
[0043] Such as figure 1 As shown, the speech emotion recognition method based on semi-supervised feature selection described in this embodiment includes two parts, the training phase and the recognition phase, now combined figure 1 The flow charts are detailed below.
[0044] 1. Training stage
[0045] In this stage, all speakers are trained separately to obtain the classifier corresponding to each speaker. The specific process is as follows:
[0046] Step 1: Extract MFCC, LFPC, LPCC, ZCPA, PLP, R for all speech training signals (for each training session, the speech signals of all labeled samples and the speech signal of a current speaker's unlabeled sample) -PLP features, where the number of Mel filters of MFCC and LFPC is 40; the linear prediction orders of LPCC, PLP, and R-PLP are 12, 16, and 16 respectively; the frequency segments of ZCPA are: 0, 106, 223, 352, 495, 655, 829, 1022, 1236, 1473, 1734, 2024, 2344, 2689, 3089, 3522, 4000. Therefore, the dimensions of each...
PUM
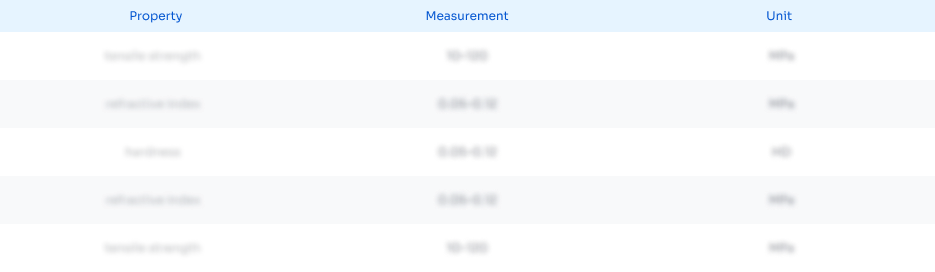
Abstract
Description
Claims
Application Information
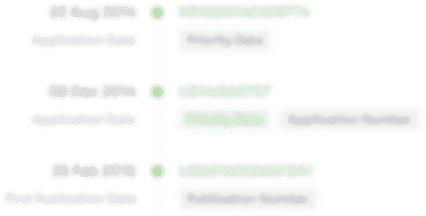
- R&D
- Intellectual Property
- Life Sciences
- Materials
- Tech Scout
- Unparalleled Data Quality
- Higher Quality Content
- 60% Fewer Hallucinations
Browse by: Latest US Patents, China's latest patents, Technical Efficacy Thesaurus, Application Domain, Technology Topic, Popular Technical Reports.
© 2025 PatSnap. All rights reserved.Legal|Privacy policy|Modern Slavery Act Transparency Statement|Sitemap|About US| Contact US: help@patsnap.com