A method of traffic identification based on bp neural network
A BP neural network and network traffic technology, applied in the field of traffic identification based on neural network, can solve problems such as inability to identify user network usage in real time, unfavorable expansion of machine learning, lack of generalization ability, etc., to achieve scalability , has the effect of realization and short time period
- Summary
- Abstract
- Description
- Claims
- Application Information
AI Technical Summary
Problems solved by technology
Method used
Image
Examples
Embodiment Construction
[0023] figure 1 It is a schematic diagram of real-time identification of network applications in the present invention. The structure in the figure is divided into two parts. Above the dividing line is the process of real-time network traffic identification, and below the dividing line is the process of synchronous sample training.
[0024] figure 2 It is the algorithm of BP neural network, the input x is the network traffic characteristic, and the network application type O is output after passing through the three-layer neural network.
[0025] image 3 (a) The picture shows the change of network flow in a time window. Taking 1 second as the time unit, the network flow captured in each second is counted, and the statistics are continuous for 15 seconds. (b) In the figure, according to the average flow rate in the time window, the flow rate in the 15-second time period is divided into a stable area and a peak area.
[0026] Offline training part of BP neural network:
[...
PUM
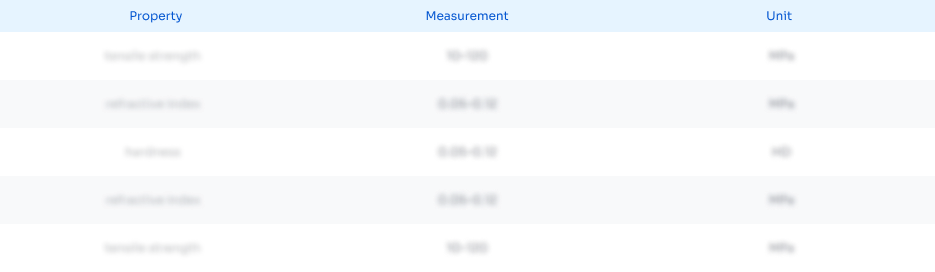
Abstract
Description
Claims
Application Information
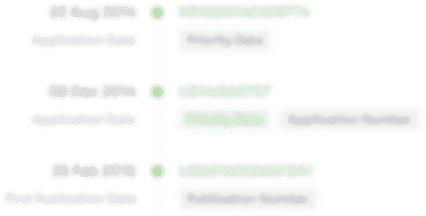
- R&D Engineer
- R&D Manager
- IP Professional
- Industry Leading Data Capabilities
- Powerful AI technology
- Patent DNA Extraction
Browse by: Latest US Patents, China's latest patents, Technical Efficacy Thesaurus, Application Domain, Technology Topic, Popular Technical Reports.
© 2024 PatSnap. All rights reserved.Legal|Privacy policy|Modern Slavery Act Transparency Statement|Sitemap|About US| Contact US: help@patsnap.com