Multi-target Fuzzy Clustering Image Change Detection Method Based on Non-Dominated Neighborhood Immune Algorithm
A technology of image change detection and immune algorithm, which is applied in the field of image processing, can solve the problems of uncertain selection of parameters, failure of the cluster center and membership matrix results by the minimum value, and influence on the accuracy of clustering results, etc., to achieve improved Stability and clustering performance, overcoming initialization sensitivity issues, and avoiding the effect of difficult parameter selection
- Summary
- Abstract
- Description
- Claims
- Application Information
AI Technical Summary
Problems solved by technology
Method used
Image
Examples
Embodiment Construction
[0033] refer to figure 1 , the specific implementation steps of the present invention are as follows:
[0034] Step 1. Given the operating parameters, set the termination condition of the algorithm.
[0035] The operating parameters include: the number of clusters K, the number of iterations T of the algorithm termination condition, the maximum algebra Gmax and the size of the antibody population Na, the mutation probability Pm, the number of clusters K, t=0, and the weighted index m of the fuzzy membership degree =2. in:
[0036] The number of clustering classes K needs to be determined according to the specific image to be processed, referring to the characteristics of the image to be segmented, and how many classes are expected to be divided into, and K is set to that number. For the difference map for change detection targeted by the present invention, there are mainly two types. That is, the change class and the change class. Therefore, K=2.
[0037] The cluster ter...
PUM
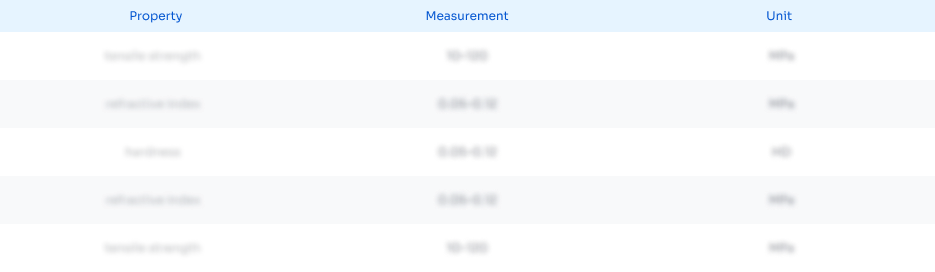
Abstract
Description
Claims
Application Information
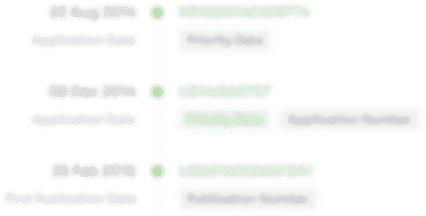
- R&D Engineer
- R&D Manager
- IP Professional
- Industry Leading Data Capabilities
- Powerful AI technology
- Patent DNA Extraction
Browse by: Latest US Patents, China's latest patents, Technical Efficacy Thesaurus, Application Domain, Technology Topic.
© 2024 PatSnap. All rights reserved.Legal|Privacy policy|Modern Slavery Act Transparency Statement|Sitemap