Longitudinal time axis clustering method in generalized load modeling on basis of seasonality
A technology of generalized load and clustering method, applied in the field of vertical time axis clustering, which can solve the problems of inability to exclude subjective factors, inability to classify all sample data, and lack of universal applicability.
- Summary
- Abstract
- Description
- Claims
- Application Information
AI Technical Summary
Problems solved by technology
Method used
Image
Examples
Embodiment Construction
[0074] The present invention is described in detail below in conjunction with accompanying drawing:
[0075] AP clustering algorithm
[0076] The traditional commonly used Bayesian classification method and distance classification method have simple models, and it is difficult to reflect the intricate relationship between seasons and load operation levels. In addition, it is difficult to cluster large sample data. In recent years, K-means clustering algorithm, fuzzy clustering algorithm, neural network method, etc. are commonly used in the classification and synthesis of load characteristics, but most of them need to be artificially set, such as the number of clusters, cluster centers, etc., and subjective factors cannot be ruled out. This application introduces the AP clustering algorithm. Affinity Propagation Clustering (AP clustering) proposed by Brendan J. Frey et al. is an effective clustering method. This method is an unsupervised clustering method. It is not necessary...
PUM
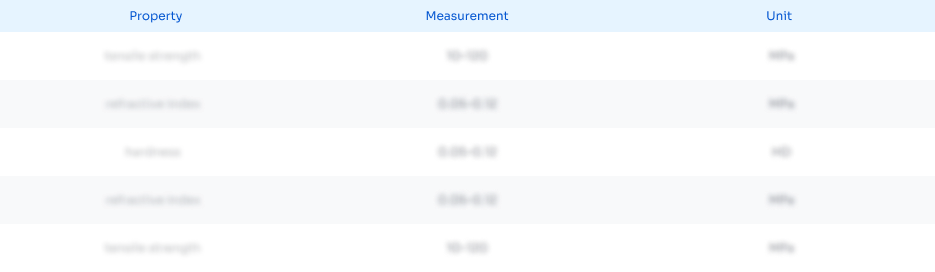
Abstract
Description
Claims
Application Information
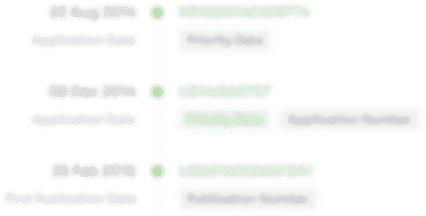
- R&D
- Intellectual Property
- Life Sciences
- Materials
- Tech Scout
- Unparalleled Data Quality
- Higher Quality Content
- 60% Fewer Hallucinations
Browse by: Latest US Patents, China's latest patents, Technical Efficacy Thesaurus, Application Domain, Technology Topic, Popular Technical Reports.
© 2025 PatSnap. All rights reserved.Legal|Privacy policy|Modern Slavery Act Transparency Statement|Sitemap|About US| Contact US: help@patsnap.com