Co-occurrence latent semantic vector space model semantic core method based on literature resource topic clustering
A technology of vector space and kernel method, which is applied in the field of semantic kernel of latent semantic vector space model of topic clustering of literature resources, which can solve problems such as high model dimension, high time and space complexity of clustering algorithm, insufficient semantic information extraction, etc. problem, achieve the effect of reducing dimensionality and improving clustering effect
- Summary
- Abstract
- Description
- Claims
- Application Information
AI Technical Summary
Problems solved by technology
Method used
Image
Examples
Embodiment 1
[0045] The first step: data preprocessing: data cleaning, labeling documents, extracting keywords of each document, and retaining the corresponding relationship between keywords and corresponding documents.
[0046] The data comes from CNKI. According to its classification, 300 documents are selected from each of the three disciplines of "Publishing", "Library Information and Digital Library" and "Archives and Museums" under the information science as the documents for analysis, except for those without keywords. There are 4 documents, and the total number of documents finally obtained is 896, including 299 articles of "publishing", 298 articles of "library information and digital library", 299 articles of "archives and museums", and 2509 different keywords were obtained. That is: the number of documents n=896, the number of keywords m=2509, the following table shows the first 20 documents and all corresponding keywords. In Table 1, LM is the document category, ID is the docum...
PUM
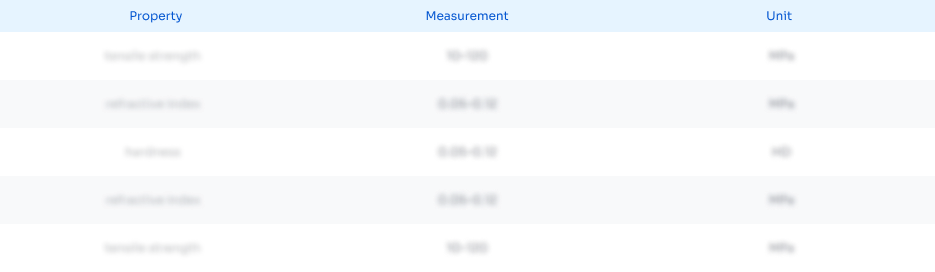
Abstract
Description
Claims
Application Information
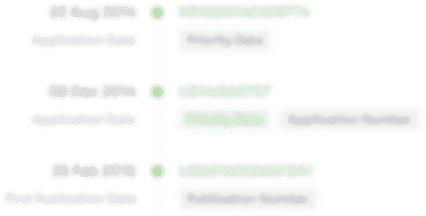
- R&D
- Intellectual Property
- Life Sciences
- Materials
- Tech Scout
- Unparalleled Data Quality
- Higher Quality Content
- 60% Fewer Hallucinations
Browse by: Latest US Patents, China's latest patents, Technical Efficacy Thesaurus, Application Domain, Technology Topic, Popular Technical Reports.
© 2025 PatSnap. All rights reserved.Legal|Privacy policy|Modern Slavery Act Transparency Statement|Sitemap|About US| Contact US: help@patsnap.com